Computed Tomography Image Segmentation of Lung Corona Virus Infection Region Based on Combination of Grayscale Morphological Reconstruction and Fast Marching Method
DOI:
https://doi.org/10.51173/jt.v5i3.1060Keywords:
Covid-19 Infection, CT Imaging, Fast Marching Method, Gray-Scale Morphological Reconstruction, Medical Image SegmentationAbstract
Recently, X-ray computed tomography-imaging modality is considered as golden standard for diagnosis of coronavirus lungs infection. In worldwide, infectious patients increase rapidly that lead to weariness in health services staff, as well as instant treatment required to avoid patients’ health deterioration due to infection development. Image processing would be reinforcing health services by considering computer-based segmentation. However, a ground glass computed tomography image fashion of coronavirus lungs infection characterized by disappearance of edge region of interest and lack of object structure. In this study, these challenges addressed by introducing a new algorithm that combined both morphological reconstruction and fast marching method. The proposed algorithm applied on archived computed tomography dataset for coronavirus infected patients, results showed consistent determination of ground glass infection region compared to manual delineation of senior physician. The proposed algorithm restricted to empirical adjustment of FMM’s threshold that would be addressed in upcoming study.
Downloads
References
P. Rai, B. K. Kumar, V. K. Deekshit, I. Karunasagar, and I. Karunasagar, "Detection technologies and recent developments in the diagnosis of COVID-19 infection," Applied Microbiology and Biotechnology, pp. 1-15, 2021.
D. Chen, X. Jiang, Y. Hong, Z. Wen, S. Wei, G. Peng, et al., "Can Chest CT Features Distinguish Patients With Negative From Those With Positive Initial RT-PCR Results for Coronavirus Disease (COVID-19)?," American Journal of Roentgenology, vol. 216, pp. 66-70, 2021/01/01 2020.
F. Shi, J. Wang, J. Shi, Z. Wu, Q. Wang, Z. Tang, et al., "Review of artificial intelligence techniques in imaging data acquisition, segmentation, and diagnosis for COVID-19," IEEE reviews in biomedical engineering, vol. 14, pp. 4-15, 2020.
M. Kim and B.-D. Lee, "Automatic lung segmentation on chest X-rays using self-attention deep neural network," Sensors, vol. 21, p. 369, 2021.
T. Agrawal and P. Choudhary, "Segmentation and classification on chest radiography: a systematic survey," The Visual Computer, pp. 1-39, 2022.
S. Pandey, P. R. Singh, and J. Tian, "An image augmentation approach using two-stage generative adversarial network for nuclei image segmentation," Biomedical Signal Processing and Control, vol. 57, p. 101782, 2020.
S. T. H. Kieu, A. Bade, M. H. A. Hijazi, and H. Kolivand, "A Survey of Deep Learning for Lung Disease Detection on Medical Images: State-of-the-Art, Taxonomy, Issues and Future Directions," Journal of Imaging, vol. 6, p. 131, 2020.
A. Oulefki, S. Agaian, T. Trongtirakul, S. Benbelkacem, D. Aouam, N. Zenati-Henda, et al., "Virtual Reality visualization for computerized COVID-19 lesion segmentation and interpretation," Biomedical Signal Processing and Control, vol. 73, p. 103371, 2022.
D.-P. Fan, T. Zhou, G.-P. Ji, Y. Zhou, G. Chen, H. Fu, et al., "Inf-net: Automatic covid-19 lung infection segmentation from ct images," IEEE Transactions on Medical Imaging, vol. 39, pp. 2626-2637, 2020.
X. Lei and H. Ouyang, "Image segmentation algorithm based on improved fuzzy clustering," Cluster Computing, vol. 22, pp. 13911-13921, 2019.
S.-M. Hou, C.-L. Jia, M.-J. Hou, S. L. Fernandes, and J.-C. Guo, "A Study on Weak Edge Detection of COVID-19’s CT Images Based on Histogram Equalization and Improved Canny Algorithm," Computational and Mathematical Methods in Medicine, vol. 2021, 2021.
R. K. Kalia, A. Sharma, S. B. Amin, M. Saha, and S. K. Thittamaranahalli, "Ai-Driven Quantification Of Ground Glass Opacities In Lungs Of Covid-19 Patients Using 3d Computed Tomography Imaging," medRxiv, 2021.
X. A. Inbaraj, C. Villavicencio, J. J. Macrohon, J.-H. Jeng, and J.-G. Hsieh, "A Novel Machine Learning Approach for Tuberculosis Segmentation and Prediction Using Chest-X-Ray (CXR) Images," Applied Sciences, vol. 11, p. 9057, 2021.
S. Chakraborty and K. Mali, "SUFMACS: a machine learning-based robust image segmentation framework for covid-19 radiological image interpretation," Expert Systems with Applications, vol. 178, p. 115069, 2021.
E. Jangam and A. Rao, "Segmentation of lungs from chest X rays using firefly optimized fuzzy C-means and level set algorithm," in International Conference on Recent Trends in Image Processing and Pattern Recognition, 2018, pp. 303-311.
J. Tan, L. Jing, Y. Huo, L. Li, O. Akin, and Y. Tian, "Lgan: Lung segmentation in ct scans using generative adversarial network," Computerized Medical Imaging and Graphics, vol. 87, p. 101817, 2021.
S. Chakraborty and K. Mali, "SuFMoFPA: A superpixel and meta-heuristic based fuzzy image segmentation approach to explicate COVID-19 radiological images," Expert Systems with Applications, vol. 167, p. 114142, 2021.
S. Chakraborty and K. Mali, "Fuzzy Electromagnetism Optimization (FEMO) and its application in biomedical image segmentation," Applied Soft Computing, vol. 97, p. 106800, 2020.
A. M. Hasan, M. M. Al-Jawad, H. A. Jalab, H. Shaiba, R. W. Ibrahim, and A. a. R. AL-Shamasneh, "Classification of Covid-19 coronavirus, pneumonia and healthy lungs in CT scans using Q-deformed entropy and deep learning features," Entropy, vol. 22, p. 517, 2020.
T. Zhou, S. Canu, and S. Ruan, "An automatic covid-19 ct segmentation based on u-net with attention mechanism," arXiv preprint arXiv:2004.06673, 2020.
K. Gao, J. Su, Z. Jiang, L.-L. Zeng, Z. Feng, H. Shen, et al., "Dual-branch combination network (DCN): Towards accurate diagnosis and lesion segmentation of COVID-19 using CT images," Medical image analysis, vol. 67, p. 101836, 2021.
M. Xu, S. Qi, Y. Yue, Y. Teng, L. Xu, Y. Yao, et al., "Segmentation of lung parenchyma in CT images using CNN trained with the clustering algorithm generated dataset," Biomedical engineering online, vol. 18, pp. 1-21, 2019.
Y. A. Hamad, M. E. Seno, M. Al-Kubaisi, and A. N. Safonova, "Segmentation and measurement of lung pathological changes for COVID-19 diagnosis based on computed tomography," Periodicals of Engineering and Natural Sciences, vol. 9, pp. 29-41, 2021.
N. Dhanachandra and Y. J. Chanu, "An image segmentation approach based on fuzzy c-means and dynamic particle swarm optimization algorithm," Multimedia Tools & Applications, vol. 79, 2020.
K. Mardani and K. Maghooli, "Enhancing retinal blood vessel segmentation in medical images using combined segmentation modes extracted by DBSCAN and morphological reconstruction," Biomedical Signal Processing and Control, vol. 69, p. 102837, 2021.
J.-W. Baek and K. Chung, "Pothole classification model using edge detection in road image," Applied Sciences, vol. 10, p. 6662, 2020.
A. N. Mathew, J. M. Kuriakose, S. Haridas, and S. John, "Study on Alzheimer’s disease using Image Processing," Journal of Embedded Systems and Processing, vol. 4.
L. Vincent, "Morphological grayscale reconstruction in image analysis: applications and efficient algorithms," IEEE transactions on image processing, vol. 2, pp. 176-201, 1993.
J. Serra, Image Analysis and Mathematical Morphology: Vol.: 2: Theoretical Advances: Academic Press, 1988.
T. Lei, X. Jia, T. Liu, S. Liu, H. Meng, and A. K. Nandi, "Adaptive morphological reconstruction for seeded image segmentation," IEEE Transactions on Image Processing, vol. 28, pp. 5510-5523, 2019.
R. D. Yapa and K. Harada, "Application of Fast-Marching method on mammograms for breast skin-line estimation," in 2007 International Conference on Industrial and Information Systems, 2007, pp. 59-62.
K. Clark, B. Vendt, K. Smith, J. Freymann, J. Kirby, P. Koppel, et al., "The Cancer Imaging Archive (TCIA): maintaining and operating a public information repository," Journal of digital imaging, vol. 26, pp. 1045-1057, 2013.
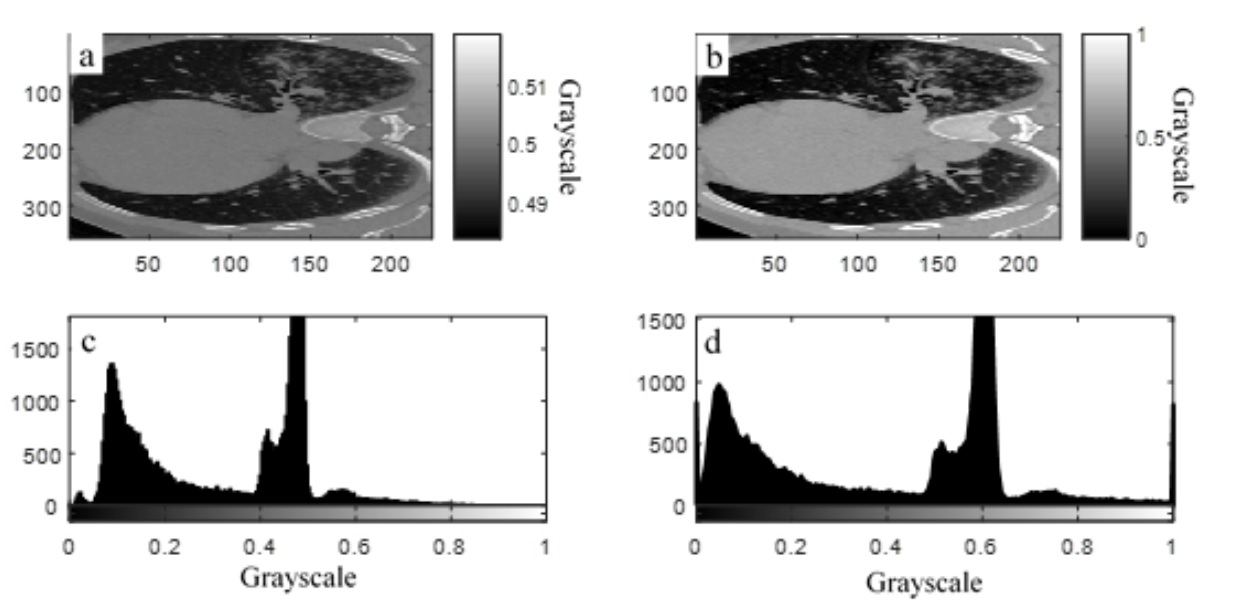
Downloads
Published
How to Cite
Issue
Section
License
Copyright (c) 2023 Aws Alazawi, Abbas Fadhal Humadi, Huda Farooq Jameel, Huda Ali Hashim, John Soraghan

This work is licensed under a Creative Commons Attribution 4.0 International License.