A Novel Rubber Composite Sleeper-Deformation-Prediction Model Based on Response Surface Method (RSM) and Machine Learning (ML) Techniques
DOI:
https://doi.org/10.51173/jt.v6i4.2609Keywords:
Rubber Composite Sleeper, Deformation, Response Surface Methodology, Machine Learning, Temperature-DistributionAbstract
Rubber composite sleepers can experience significant temperature variations in service, causing temperature-induced deformation. Real-time monitoring of this deformation is crucial for operational safety and maintenance; however, it is costly, time-consuming, and requires substantial resources and personnel.
Developing temperature-dependent predictive models offers a cost-effective and efficient alternative, providing accurate insights into sleeper behaviour under different conditions while saving time, labour, and materials. This study attempts to develop a novel deformation model of rubber composite sleepers using response surface methodology (RSM) and machine learning (ML) techniques. Platinum temperature (Pt) sensors, embedded at various points on a full-scale rubber composite sleeper model, were used to measure both the sleeper temperature field and ambient temperature in real-time at 30-minute intervals over the period of a year. Simultaneously, lateral deformation was recorded using linear variable differential transducer (LVDT) displacement sensors. The temperature data were filtered to remove noise and normalized based on the Log-Pearson Type III outlier detection method and Box-Cox transformation, respectively, before being used to develop temperature-dependent models for sleeper deformation. To ensure accurate ML predictions, the dataset was split into 70% for training and 30% for testing. Model performance was evaluated using the correlation coefficient (R2), mean square error (MSE), root means square error (RMSE), and mean absolute error (MAE). The analysis revealed that the sleeper’s body temperature closely follows the changing trend of the ambient environment. Also, like any polymer material, the rubber composite sleeper expands when it absorbs heat from sunlight and contracts as it cools when sunlight intensity decreases, potentially reversing much of the deformation. The K-nearest neighbour algorithm outperformed the RSM and other ML techniques with R2, MSE, RMSE, and MAE values of 0.999, 0.000258, 0.016, and 0.000896, respectively. The developed model can serve as an important reference for monitoring lateral deformation for safety and maintenance purposes.
Downloads
References
É. A. Silva, D. Pokropski, R. You, and S. Kaewunruen, "Comparison of structural design methods for railway composites and plastic sleepers and bearers," Australian journal of structural engineering, vol. 18, no. 3, pp. 160-177, 2017. https://doi.org/10.1080/13287982.2017.1382045.
W. Ferdous, A. Manalo, T. Aravinthan, and A. Remennikov, "Recent developments and applications of composite railway sleepers," CORE 2016: Maintaining the Momentum, p. 197, 2016.https://search.informit.org/doi/10.3316/informit.428799011790553.
A. Ghorbani and S. Erden, "Polymeric composite railway sleepers," Sci. Rep, vol. 6, pp. 9-11, 2013.
W. Ferdous, T. Aravinthan, A. Manalo, and G. Van Erp, "Composite railway sleepers: New developments and opportunities," in Proceedings of the 11th International Heavy Haul Association Conference (IHHA 2015), 2015: International Heavy Haul Association, pp. 1-9.
I. Lotfy, M. Farhat, M. A. Issa, and M. Al-Obaidi, "Flexural behavior of high-density polyethylene railroad crossties," Proceedings of the Institution of Mechanical Engineers, Part F: Journal of Rail and Rapid Transit, vol. 230, no. 3, pp. 813-824, 2016, https://doi.org/10.1177/0954409714565655.
W. Ferdous and A. Manalo, "Failures of mainline railway sleepers and suggested remedies–review of current practice," Engineering Failure Analysis, vol. 44, pp. 17-35, 2014, https://doi.org/10.1016/j.engfailanal.2014.04.020.
Y. Pang, S. N. Lingamanaik, B. K. Chen, and S. F. Yu, "Measurement of deformation of the concrete sleepers under different support conditions using non-contact laser speckle imaging sensor," Engineering Structures, vol. 205, p. 110054, 2020/02/15/ 2020, doi: https://doi.org/10.1016/j.engstruct.2019.110054, https://doi.org/10.1016/j.engstruct.2019.110054.
S. Kaewunruen, R. You, and M. Ishida, "Composites for timber-replacement bearers in railway switches and crossings," Infrastructures, vol. 2, no. 4, p. 13, 2017, https://doi.org/10.3390/infrastructures2040013.
V. I. Kondrashchenko, G. Q. Jing, and C. Wang, "Wood-polymer composite for the manufacture of sleepers," in Materials Science Forum, 2019, vol. 945: Trans Tech Publ, pp. 509-514, https://doi.org/10.4028/www.scientific.net/MSF.945.509.
W. Ferdous, A. Manalo, G. Van Erp, T. Aravinthan, S. Kaewunruen, and A. Remennikov, "Composite railway sleepers–Recent developments, challenges and future prospects," Composite Structures, vol. 134, pp. 158-168, 2015, https://doi.org/10.1016/j.compstruct.2015.08.058.
E. Ferro, J. Harkness, and L. Le Pen, "The influence of sleeper material characteristics on railway track behaviour: concrete vs composite sleeper," Transportation Geotechnics, vol. 23, p. 100348, 2020, https://doi.org/10.1016/j.trgeo.2020.100348.
G. Van Erp and M. Mckay, "Recent Australian developments in fibre composite railway sleepers," Electronic Journal of Structural Engineering, vol. 13, no. 1, pp. 62-6, 2013, https://doi.org/10.56748/ejse.131611.
E. R. Anne, "Rubber/plastic composite rail sleepers," UK: The waste & resources action programme, 2006.
P. Chow, "Test report on mechanical properties with eight integrico composite crossties," Urbana, USA: University of Illinois, 2007.
P. Cromberge, "Polymer rail sleepers being tested for the mining industry," Mining Weekly, 2005.
C. Pattamaprom, D. Dechojarassri, C. Sirisinha, and W. Kanok-Nukulchai, "Natural rubber composites for railway sleepers: A feasibility study," Thammasat University, Thailand, 2005.
G. Graebe, J. Woidasky, and J. Fraunhofer, "Railway sleepers from mixed plastic waste-Railwaste project status information," Fraunhofer ICT, 2010.
S. Kaewunruen, "Acoustic and dynamic characteristics of a complex urban turnout using fibre-reinforced foamed urethane (FFU) bearers," in Noise and Vibration Mitigation for Rail Transportation Systems: Springer, 2015, pp. 377-384, https://doi.org/10.1007/978-3-662-44832-8_45.
W. Ferdous et al., "Composites for alternative railway sleepers," in EASEC16: Springer, 2021, pp. 267-276, https://doi.org/10.1007/978-981-15-8079-6_26.
A. S. Shanour, A. A. Khalil, H. S. Riad, and H. M. Bakry, "Experimental and analytical investigations of innovative composite materials using GFRP and iron slag for railway sleepers," J. Eng. Res. Reports, pp. 25-42, 2020.
Z. Zeng, A. A. Shuaibu, F. Liu, M. Ye, and W. Wang, "Experimental study on the vibration reduction characteristics of the ballasted track with rubber composite sleepers," Construction and Building Materials, vol. 262, p. 120766, 2020, https://doi.org/10.1016/j.conbuildmat.2020.120766.
Z. Zeng, A. A. Qahtan, G. Hu, R. Xu, and A. A. Shuaibu, "Comparative experimental investigation of the vibration mitigation characteristics of ballasted track using the rubber composite sleeper and concrete sleeper under various interaction forces," Engineering Structures, vol. 275, p. 115243, 2023, https://doi.org/10.1016/j.engstruct.2022.115243.
Zeng, Z., Ye, M., Wang, W., Liu, J., Shen, S. and Qahtan, A.A.S., 2022. Analysis on mechanical characteristics of CRTSII slab ballastless track structures in rectification considering material brittleness. Construction and Building Materials, 319, p.126058, https://doi.org/10.1016/j.conbuildmat.2021.126058.
Z. Zhao, Y. Gao, and C. Li, "Research on the Vibration Characteristics of a Track’s Structure Considering the Viscoelastic Properties of Recycled Composite Sleepers," Applied Sciences, vol. 11, no. 1, p. 150, 2021, https://doi.org/10.3390/app11010150.
Zhao, Z., Shen, Y., Wei, Q., Jiang, W., Geng, H. and Li, C., 2019. Experimental study on dynamic performance of composite sleeper ballasted track. J. Cent. South Univ. Sci. Technol, 50, pp.234-240, doi: 10.11817/j.issn.1672-7207.2019.01.029.
M. A. Jabu, A. Alugongo, and N. Nkomo, "An Experimental Investigation on Effect of Rubber Particle Size on Composite Railway Sleeper Mechanical Strength and Vibrational Damping Properties.", International Journal of Engineering Trends and Technology, 72(2), 222-229, February 2024, https://doi.org/10.14445/22315381/IJETT-V72I2P123.
Z. Zhao, Y. Gao, and C. Li, "Experimental Study on Dynamic Properties of a Recycled Composite Sleeper and Its Theoretical Model," Symmetry, vol. 13, no. 1, p. 17, 2021, https://doi.org/10.3390/sym13010017.
V. Lojda, A. van Belkom, and H. Krejčiříková, "Investigation of the elastic modulus of polymer sleepers under a quasistatic and cyclic loading," Civil and Environmental Engineering, vol. 15, no. 2, pp. 125-133, 2019, DOI: 10.2478/cee-2019-0016.
I. Lotfy, M. Farhat, and M. A. Issa, "Effect of pre-drilling, loading rate and temperature variation on the behavior of railroad spikes used for high-density-polyethylene crossties," Proceedings of the Institution of Mechanical Engineers, Part F: Journal of Rail and Rapid Transit, vol. 231, no. 1, pp. 44-56, 2017, https://doi.org/10.1177/0954409715620755.
P. Yu et al., "Investigation on the physical, mechanical and microstructural properties of epoxy polymer matrix with crumb rubber and short fibres for composite railway sleepers," Construction and Building Materials, vol. 295, p. 123700, 2021, https://doi.org/10.1016/j.conbuildmat.2021.123700.
M. Siahkouhi, X. Li, V. L. Markine, and G. Jing, "Experimental and numerical study on the mechanical behavior of Kunststof Lankhorst Product (KLP) sleepers," Scientia Iranica, 28(5), pp.2568-2581, 2021, https://doi.org/10.24200/sci.2021.57165.5096.
C. Salih et al., "Novel Bending Test Method for Polymer Railway Sleeper Materials," Polymers, vol. 13, 2021, https://doi.org/10.3390/polym13091359.
Z. Zeng, Z. Huang, H. Yin, X. Meng, W. Wang, and J. Wang, "Influence of track line environment on the temperature field of a double-block ballastless track slab," Advances in Mechanical Engineering, vol. 10, no. 12, p. 1687814018812325, 2018, https://doi.org/10.1177/1687814018812325.
X. Chen, Y. Zhang, and P. Ge, "Prediction of concrete strength using response surface function modified depth neural network," (in eng), PloS one, vol. 18, no. 5, p. e0285746, 2023, doi: 10.1371/journal.pone.0285746.
T. Awolusi, O. Oke, O. Akinkurolere, and A. Sojobi, "Application of response surface methodology: Predicting and optimizing the properties of concrete containing steel fibre extracted from waste tires with limestone powder as filler," Case studies in Construction materials, vol. 10, p. e00212, 2019, https://doi.org/10.1016/j.cscm.2018.e00212.
M. H. Osman et al., "Response surface methodology optimization of concrete strength using hydroxyapatite nanopowder as admixture," Progress in Engineering Application and Technology, vol. 1, no. 1, pp. 134-141, 2020.
D. Sinkhonde, R. O. Onchiri, W. O. Oyawa, and J. N. Mwero, "Response surface methodology-based optimisation of cost and compressive strength of rubberised concrete incorporating burnt clay brick powder," Heliyon, vol. 7, no. 12, p. e08565, 2021,https://doi.org/10.1016/j.heliyon.2021.e08565.
I. K. Harith, M. J. Hussein, and M. S. Hashim, "Optimization of the synergistic effect of micro silica and fly ash on the behavior of concrete using response surface method," Open Engineering, vol. 12, no. 1, pp. 923-932, 2022, https://doi.org/10.1515/eng-2022-0332.
S. A. Nwose, F. Edoziuno, and S. Osuji, "Statistical analysis and Response Surface Modelling of the compressive strength inhibition of crude oil in concrete test cubes," Algerian Journal of Engineering and Technology, vol. 4, pp. 99-107, 2021, http://dx.doi.org/10.5281/zenodo.4696030.
Y. Gong, J. Song, S. Lin, J. Yang, Y. He, and G. Tan, "Design optimization of rubber-basalt fiber-modified concrete mix ratios based on a response surface method," Applied Sciences, vol. 10, no. 19, p. 6753, 2020, https://doi.org/10.3390/app10196753.
Sinkhonde, David, Richard Ocharo Onchiri, Walter Odhiambo Oyawa, and John Nyiro Mwero. "Behaviour of rubberised concrete with waste clay brick powder under varying curing conditions." Heliyon, 9(2), (2023, https://doi.org/10.1016/j.heliyon.2023.e13372.
A. Usman, M. H. Sutanto, M. Napiah, S. E. Zoorob, N. S. A. Yaro, and M. I. Khan, "Comparison of performance properties and prediction of regular and gamma-irradiated granular waste polyethylene terephthalate modified asphalt mixtures," Polymers, vol. 13, no. 16, p. 2610, 2021, https://doi.org/10.3390/polym13162610.
N. S. A. Yaro et al., "Modeling and optimization of rheological properties and aging resistance of asphalt binder incorporating palm oil mill waste using response surface methodology," Journal of Infrastructure Intelligence and Resilience, vol. 2, no. 1, p. 100026, 2023, https://doi.org/10.1016/j.iintel.2023.100026.
T. B. Moghaddam, M. Soltani, M. R. Karim, and H. Baaj, "Optimization of asphalt and modifier contents for polyethylene terephthalate modified asphalt mixtures using response surface methodology," Measurement, vol. 74, pp. 159-169, 2015, https://doi.org/10.1016/j.measurement.2015.07.012.
A. Rema and A. K. Swamy, "Response Surface–Based Approach to Quantify Variability in Recycled-Asphalt Concrete Mixtures," Journal of Materials in Civil Engineering, vol. 34, no. 11, p. 04022315, 2022, https://doi.org/10.1061/(ASCE)MT.1943-5533.0004479.
J. Pagaimo, H. Magalhães, J. Costa, and J. Ambrosio, "Derailment study of railway cargo vehicles using a response surface methodology," Vehicle System Dynamics, vol. 60, no. 1, pp. 309-334, 2022, https://doi.org/10.1080/00423114.2020.1815810.
J. Wang, S. Chen, X. Li, and Y. Wu, "Optimal rail profile design for a curved segment of a heavy haul railway using a response surface approach," Proceedings of the Institution of Mechanical Engineers, Part F: Journal of Rail and Rapid Transit, vol. 230, no. 6, pp. 1496-1508, 2016/08/01 2015, doi: 10.1177/0954409715602513.
Shi, J., Gao, Y., Long, X. and Wang, Y., Optimizing rail profiles to improve metro vehicle-rail dynamic performance considering worn wheel profiles and curved tracks. Structural and Multidisciplinary Optimization, 63, pp.419-438, 2021, https://doi.org/10.1007/s00158-020-02680-7.
S. Liu et al., "Deformation Analysis and Prediction of a High-Speed Railway Suspension Bridge under Multi-Load Coupling," Remote Sensing, vol. 16, no. 10, p. 1687, 2024, https://doi.org/10.3390/rs16101687.
A. Ramos, A. Gomes Correia, K. Nasrollahi, J. C. O. Nielsen, and R. Calçada, "Machine Learning Models for Predicting Permanent Deformation in Railway Tracks," Transportation Geotechnics, vol. 47, p. 101289, 2024/07/01/ 2024, doi: https://doi.org/10.1016/j.trgeo.2024.101289.
J. Chen et al., "A deep learning forecasting method for frost heave deformation of high-speed railway subgrade," Cold Regions Science and Technology, vol. 185, p. 103265, 2021/05/01/ 2021, doi: https://doi.org/10.1016/j.coldregions.2021.103265.
S. Kaewunruen, J. Sresakoolchai, J. Huang, Y. Zhu, C. Ngamkhanong, and A. M. Remennikov, "Machine Learning Based Design of Railway Prestressed Concrete Sleepers," Applied Sciences, vol. 12, no. 20, p. 10311, 2022, https://doi.org/10.3390/app122010311.
S. Hong, C. Park, and S. Cho, "A rail-temperature-prediction model based on machine learning: warning of train-speed restrictions using weather forecasting," Sensors, vol. 21, no. 13, p. 4606, 2021, https://doi.org/10.3390/s21134606.
B. Indraratna, D. J. Armaghani, A. Gomes Correia, H. Hunt, and T. Ngo, "Prediction of resilient modulus of ballast under cyclic loading using machine learning techniques," Transportation Geotechnics, vol. 38, p. 100895, 2023/01/01/ 2023, doi: https://doi.org/10.1016/j.trgeo.2022.100895.
P. Aela, J. Wang, K. Yousefian, H. Fu, Z.-Y. Yin, and G. Jing, "Prediction of crushed numbers and sizes of ballast particles after breakage using machine learning techniques," Construction and Building Materials, vol. 337, p. 127469, 2022, https://doi.org/10.1016/j.conbuildmat.2022.127469.
S. Kako, "A Comparative Study about Accuracy Levels of Resistance Temperature Detectors RTDs Composed of Platinum, Copper, and Nickel," Al-Nahrain Journal for Engineering Sciences, vol. 26, pp. 216-225, 10/31 2023, doi: 10.29194/NJES.26030216.
S. Hong et al., "Prediction of a representative point for rail temperature measurement by considering longitudinal deformation," Proceedings of the Institution of Mechanical Engineers, Part F: Journal of Rail and Rapid Transit, vol. 233, no. 10, pp. 1003-1011, 2019, https://doi.org/10.1177/0954409718822866.
Fang, Xiu-Yang, Jian-En Gong, Feng Zhang, Hao-Nan Zhang, and Jia-Hong Wu. "Machine learning assisted materials design of high-speed railway wheel with better fatigue performance." Engineering Fracture Mechanics 292, 109586, 2023, https://doi.org/10.1016/j.engfracmech.2023.109586.
I. Reis, D. Baron, and S. Shahaf, "Probabilistic random forest: A machine learning algorithm for noisy data sets," The Astronomical Journal, vol. 157, no. 1, p. 16, 2018, DOI 10.3847/1538-3881/aaf101.
M. Savargiv, B. Masoumi, and M. R. Keyvanpour, "A new random forest algorithm based on learning automata," Computational Intelligence and Neuroscience, vol. 2021, 2021, https://doi.org/10.1155/2021/5572781.
S. Hong et al., "Prediction of a representative point for rail temperature measurement by considering longitudinal deformation," Proceedings of the Institution of Mechanical Engineers, Part F: Journal of Rail and Rapid Transit, vol. 233, p. 095440971882286, 03/06 2019, doi: 10.1177/0954409718822866.
Dang, Thanh Kim Mai, Mostafa Nikzad, Reza Arablouei, Syed Masood, Dac-Khuong Bui, Vi Khanh Truong, and Igor Sbarski. "Experimental study and predictive modelling of damping ratio in hybrid polymer concrete." Construction and Building Materials 411, 134541, 2024, https://doi.org/10.1016/j.conbuildmat.2023.134541.
C. Lee and S. Lee, "Exploring the Contributions by Transportation Features to Urban Economy: An Experiment of a Scalable Tree-Boosting Algorithm with Big Data," Land, vol. 11, no. 4, p. 577, 2022. https://doi.org/10.3390/land11040577.
T. Chen and C. Guestrin, "Xgboost: A scalable tree boosting system," in Proceedings of the 22nd acm sigkdd international conference on knowledge discovery and data mining, pp. 785-794, 2016, https://doi.org/10.1145/2939672.2939785.
E. Okafor, D. O. Obada, Y. Ibrahim, and D. Dodoo‐Arhin, "Prediction of the reflection intensity of natural hydroxyapatite using generalized linear model and ensemble learning methods," Engineering Reports, vol. 3, no. 2, p. e12292, 2021, https://doi.org/10.1002/eng2.12292.
M. Kamran, B. Ullah, M. Ahmad, and M. M. S. Sabri, "Application of KNN-based isometric mapping and fuzzy c-means algorithm to predict short-term rockburst risk in deep underground projects," Frontiers in Public Health, vol. 10, p. 1023890, 2022, doi: 10.3389/fpubh.2022.1023890.
P. T. Nguyen, "Application machine learning in construction management," TEM Journal, vol. 10, no. 3, pp. 1385-1389, 2021.
S. C. Chelgani, H. Nasiri, A. Tohry, and H. Heidari, "Modeling industrial hydrocyclone operational variables by SHAP-CatBoost-A “conscious lab” approach," Powder Technology, vol. 420, p. 118416, 2023, https://doi.org/10.1016/j.powtec.2023.118416.
Ahmad, Ayaz, Krzysztof Adam Ostrowski, Mariusz Maślak, Furqan Farooq, Imran Mehmood, and Afnan Nafees. "Comparative study of supervised machine learning algorithms for predicting the compressive strength of concrete at high temperature." Materials 14(15), 4222, 2021, https://doi.org/10.3390/ma14154222.
J. T. Hancock and T. M. Khoshgoftaar, "CatBoost for big data: an interdisciplinary review," Journal of Big Data, vol. 7, no. 1, p. 94, 2020/11/04 2020, doi: 10.1186/s40537-020-00369-8.
L. Prokhorenkova, G. Gusev, A. Vorobev, A. V. Dorogush, and A. Gulin, "CatBoost: unbiased boosting with categorical features," Advances in neural information processing systems, vol. 31, 2018.
N. M. Shahani, M. Kamran, X. Zheng, C. Liu, and X. Guo, "Application of Gradient Boosting Machine Learning Algorithms to Predict Uniaxial Compressive Strength of Soft Sedimentary Rocks at Thar Coalfield," Advances in Civil Engineering, vol. 2021, p. 2565488, 2021/11/01 2021, doi: 10.1155/2021/2565488.
V. Rathakrishnan, S. Bt. Beddu, and A. N. Ahmed, "Predicting compressive strength of high-performance concrete with high volume ground granulated blast-furnace slag replacement using boosting machine learning algorithms," Scientific Reports, vol. 12, no. 1, p. 9539, 2022/06/09 2022, doi: 10.1038/s41598-022-12890-2.
D. Mohammadzadeh S, S.-F. Kazemi, A. Mosavi, E. Nasseralshariati, and J. H. Tah, "Prediction of compression index of fine-grained soils using a gene expression programming model," Infrastructures, vol. 4, no. 2, p. 26, 2019, https://doi.org/10.3390/infrastructures4020026.
M. Hajihassani, S. S. Abdullah, P. G. Asteris, and D. J. Armaghani, "A gene expression programming model for predicting tunnel convergence," Applied Sciences, vol. 9, no. 21, p. 4650, 2019, https://doi.org/10.3390/app9214650.
M. F. Javed et al., "Applications of gene expression programming and regression techniques for estimating compressive strength of bagasse ash based concrete," Crystals, vol. 10, no. 9, p. 737, 2020, https://doi.org/10.3390/cryst10090737.
F. Aslam et al., "Applications of gene expression programming for estimating compressive strength of high-strength concrete," Advances in Civil Engineering, vol. 2020, 2020, https://doi.org/10.1155/2020/8850535.
S. Abdulrazaq, "Streamflow prediction in ungauged river basin using gene expression programming," Universiti Teknologi Malaysia, 2016.
T. Chai and R. R. Draxler, "Root mean square error (RMSE) or mean absolute error (MAE)?–Arguments against avoiding RMSE in the literature," Geoscientific model development, vol. 7, no. 3, pp. 1247-1250, 2014, https://doi.org/10.5194/gmd-7-1247-2014.
T. O. Hodson, "Root mean square error (RMSE) or mean absolute error (MAE): when to use them or not," Geoscientific Model Development Discussions, pp. 1-10, 2022, https://doi.org/10.5194/gmd-15-5481-2022.
D. S. K. Karunasingha, "Root mean square error or mean absolute error? Use their ratio as well," Information Sciences, vol. 585, pp. 609-629, 2022/03/01/ 2022, doi: https://doi.org/10.1016/j.ins.2021.11.036.
W. Wang and Y. Lu, "Analysis of the mean absolute error (MAE) and the root mean square error (RMSE) in assessing rounding model," in IOP conference series: materials science and engineering, 2018, vol. 324, no. 1: IOP Publishing, p. 012049, DOI 10.1088/1757-899X/324/1/012049.
A. H. Gandomi, S. K. Babanajad, A. H. Alavi, and Y. Farnam, "Novel approach to strength modeling of concrete under triaxial compression," Journal of materials in civil engineering, vol. 24, no. 9, pp. 1132-1143, 2012, https://doi.org/10.1061/(ASCE)MT.1943-5533.0000494.
A. Garcia Asuero, A. Sayago, and G. González, "The Correlation Coefficient: An Overview," Critical Reviews in Analytical Chemistry - CRIT REV ANAL CHEM, vol. 36, pp. 41-59, 01/01 2006, doi: 10.1080/10408340500526766.
Sammut, Claude, and Geoffrey I. Webb, eds. Encyclopedia of machine learning. Springer Science & Business Media, 2011.
Schneider, Patrick, and Fatos Xhafa. Anomaly detection and complex event processing over iot data streams: with application to EHealth and patient data monitoring. Academic Press, 2022.
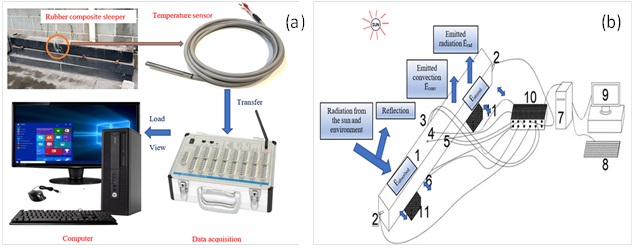
Downloads
Published
How to Cite
Issue
Section
License
Copyright (c) 2024 Abdulmumin Ahmed Shuaibu, Zhiping Zeng, Ibrahim Hayatu Hassan, Wang Weidong, Hassan Suleiman Otuoze, Suleiman Abdulhakeem, Bushrah Baba Abdulrahman

This work is licensed under a Creative Commons Attribution 4.0 International License.