The Impact of (DEM) Accuracy and (LC/LU) Resolution on the Watersheds Areas
DOI:
https://doi.org/10.51173/jt.v4i1.437Keywords:
Land Cover/Land Use, Watershed Delineation, Remote Sensing Satellites, Geographic Information System, Digital Elevation ModelAbstract
Land Cover/Land Use (LC/LU) and Digital Elevation Model (DEM) are the main inputs for watershed modelling. Recently, (DEM) and (LC/LU) are freely available as online open-source products in varied accuracies, spatial and spectral resolutions as result of several remote sensing platforms. Therefore, it is very important to determine which one is the optimum for modeling the watersheds in the selected study area, which is represented by five valley watersheds of diverse characteristics, located on east and west sides of the Mosul dam reservoir, Nineveh province, Iraq. In this research, the different accuracy of 30m resolution DEMs with Satellites (Copernicus (GLO-30), ASTER, and SRTM), besides to supervised classification (Support vector machines (SVM) classifier) results (LC/LU) main layers (green land, bare soil, urban areas, and water) of different spatial and spectral resolutions images with Satellites (10m Sentinel-2, 30m Landsat-8, and resampled 15m from 30m Landsat-8) are examined by using the techniques of Remote Sensing (RS) and Geographic Information System (GIS). Analysis of the results led to the finding that Copernicus DEM (GLO-30) 30 m spatial resolution is the most accurate and optimum DEM in the research study area with 1.1615 m vertical accuracy and 2.276 m at 95% confidence level. The optimum most accurate image for (LC/LU) thematic map production in the selected area is (Sentinel-2 l0 m) satellite at overall Classification accuracy (97%) and the overall kappa statistics (95%). The optimum remote sensing data (sentinel-2 image and Copernicus DEM) are mapped, 3D simulated and analyzed by GIS to calculate the areas of (LC/LU) main layers and to find that the considerable part of the selected watersheds is the area of green lands 404.32676 km2 was about 54.396% of the total area in the study, and these areas are vary depending on many factors such as remote sensing data, precipitation, cultivation, season, and human activities.
Downloads
References
R. Palaka and G. J. Sankar, “Study of watershed characteristics using Google Elevation Service,” in Geospatial world, 2016, no. March, doi: 10.13140/2.1.5103.0080.
J. Kim, J. Noh, K. Son, and I. Kim, “Impacts of GIS data quality on determination of runoff and suspended sediments in the Imha watershed in Korea,” Geosci. J., vol. 16, no. 2, pp. 181–192, 2012, doi: 10.1007/s12303-012-0013-8.
C. C. Mbajiorgu, K. N. Ogbu, and V. Ogwo, “IMPACT OF DEM RESOLUTION ON WATERSHED TOPOGRAPHIC INDICES AND SIMULATED HYDROLOGIC MODELING RESULTS,” no. September, 2015.
J. Thomas, V. Prasannakumar, and P. Vineetha, “Suitability of spaceborne digital elevation models of different scales in topographic analysis: an example from Kerala, India,” Environ. Earth Sci., vol. 73, no. 3, pp. 1245–1263, 2015, doi: 10.1007/s12665-014-3478-0.
R. A. Schowengerdt, Remote Sensing: Models and Methods for Image Processing, 3rd ed. Elsevier Inc., 2007.
P. F. Hsieh, L. C. Lee, and N. Y. Chen, “Effect of spatial resolution on classification errors of pure and mixed pixels in remote sensing,” IEEE Trans. Geosci. Remote Sens., vol. 39, no. 12, pp. 2657–2663, 2001, doi: 10.1109/36.975000.
F. H. Saeed and M. S. Al-Khafaji, “Assessing the Accuracy of Runoff Modelling with Different Spectral and Spatial Resolution Data Using SWAT Model.” Thesis submitted to the building and construction engineering Department, University of Technology, 2016.
M. E. Mohammad, N. Al-Ansari, I. E. Issa, and S. Knutsson, “Sediment in Mosul Dam reservoir using the HEC-RAS model,” Lakes Reserv. Res. Manag., vol. 21, no. 3, pp. 235–244, 2016, doi: 10.1111/lre.12142.
IAMAT, “Iraq Climate Data,” 24 world climate and food safety charts, 2020. https://www.iamat.org/country/iraq/climate-data (accessed Dec. 30, 2020).
M. E. Mohammad, N. A. Al-Ansari, and S. Knutsson, “Sediment delivery from right bank valleys to Mosul Reservoir Iraq,” J. Ecol. Environ. Sci., vol. 3, no. 1, pp. 50–53, 2012.
N. Al-ansari and S. Knutsson, “Toward Prudent management of Water Resources in Iraq,” J. Adv. Sci. Eng. Res., vol. 1, pp. 53–67, 2011.
ECB, “Sedimentation study at the intake of North Al- Jazeera Irrigation project. Final report,” Eng. Consult. Bur., p. 112, 2010.
W. Shi, B. Wang, and Y. Tian, “Accuracy Analysis of Digital Elevation Model Relating to Spatial Resolution and Terrain Slope by Bilinear Interpolation,” Math. Geosci., vol. 46, no. 4, pp. 445–481, 2014, doi: 10.1007/s11004-013-9508-8.
W. Katerji, M. F. Abadia, and M. D. C. M. Balsera, “Dem Local Accuracy Patterns in Land-Use/Land-Cover Classification,” Open Geosci., vol. 8, no. 1, pp. 760–770, 2016, doi: 10.1515/geo-2016-0052.
C. Suwanprasit and N. Srichai, “Impacts of spatial resolution on land cover classification,” Proc. Asia-Pacific Adv. Netw., vol. 33, no. 0, p. 39, 2012, doi: 10.7125/apan.33.4.
Y. Yanjun, T. Qingjiu, Z. Yulin, T. Bo, and X. Kaijian, “Effects of Spatial Resolution and Texture Features on Multi-spectral Remote Sensing Classification,” Geo-information Sci., vol. 20, no. 1, pp. 99–107, 2018, doi: 10.12082/dqxxkx.2018.170177.
L. Chasmer, C. Hopkinson, T. Veness, W. Quinton, and J. Baltzer, “A decision-tree classi fi cation for low-lying complex land cover types within the zone of discontinuous permafrost,” Remote Sens. Environ., vol. 143, pp. 73–84, 2014, doi: 10.1016/j.rse.2013.12.016.
T. Blaschke, “Object based image analysis for remote sensing,” ISPRS J. Photogramm. Remote Sens., vol. 65, no. 1, pp. 2–16, 2010, doi: 10.1016/j.isprsjprs.2009.06.004.
N. Dey, A. S. Ashour, and C. Bhatt, Eds., Big Data for Remote Sensing: Visualization, Analysis and Interpretation. Springer, 2019.
A. Razaque, M. Ben Haj Frej, M. Almi’ani, M. Alotaibi, and B. Alotaibi, “Improved support vector machine enabled radial basis function and linear variants for remote sensing image classification,” Sensors, vol. 21, no. 13, pp. 1–26, 2021, doi: 10.3390/s21134431.
B. Rimal, S. Rijal, and R. Kunwar, “Comparing Support Vector Machines and Maximum Likelihood Classifiers for Mapping of Urbanization,” J. Indian Soc. Remote Sens., vol. 48, no. 1, pp. 71–79, 2020, doi: 10.1007/s12524-019-01056-9.
A. Mondal, S. Kundu, S. K. Chandniha, R. Shukla, and P. K. Mishra, “Comparison of Support Vector Machine and Maximum Likelihood Classification Technique using Satellite Imagery,” vol. 1, no. 2, pp. 116–123, 2012.
C. H. Grohmann, “Evaluation of TanDEM-X DEMs on selected Brazilian sites: Comparison with SRTM, ASTER GDEM and ALOS AW3D30,” Remote Sens. Environ., vol. 212, no. September 2017, pp. 121–133, 2018, doi: 10.1016/j.rse.2018.04.043.
P. L. Guth and T. M. Geoffroy, “LiDAR point cloud and ICESat-2 evaluation of 1 second global digital elevation models: Copernicus wins,” Trans. GIS, vol. 25, no. 5, pp. 2245–2261, 2021, doi: 10.1111/tgis.12825.
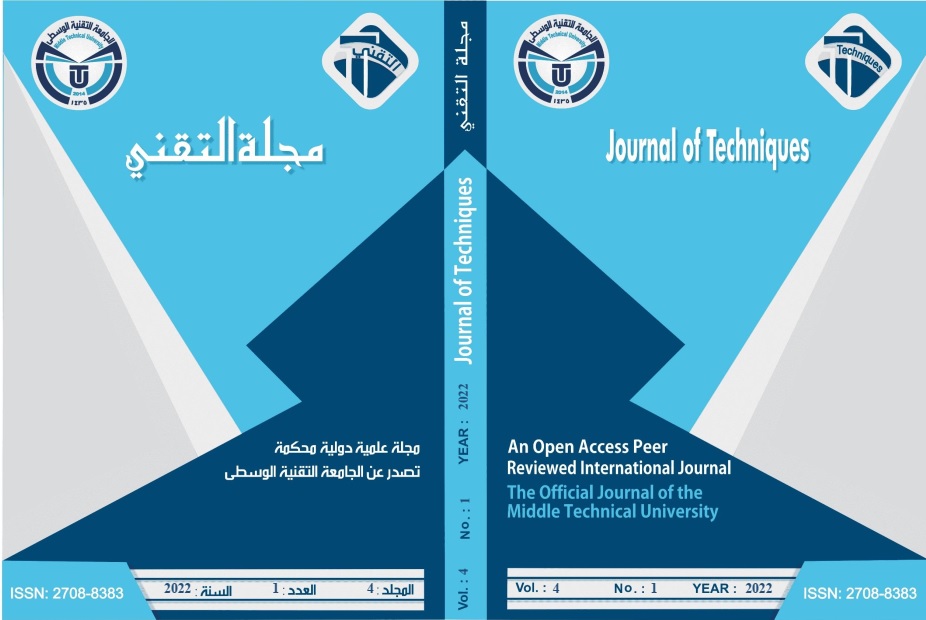
Downloads
Published
How to Cite
Issue
Section
License
Copyright (c) 2022 Alaa Qais Muttar, Mustafa Tariq Mustafa, Muntasir Abdl Hameed Abdl Shareef

This work is licensed under a Creative Commons Attribution 4.0 International License.