CT-Scan Method-based Artificial Neural Network for Diagnosis of COVID-19
DOI:
https://doi.org/10.51173/jt.v4i4.701Keywords:
ANN, Backpropagation Neural Network, COVID-19, CT-Scan, D-Dimer Diagnosis, Heart Rate, SpO2, X-rayAbstract
The Covid-19 epidemic appeared suddenly, with a rapid start and leaping steps, declaring a threat to global health where it was the beginnings of its upbringing in Wuhan, China. Where the World Health Organization announced after confirming the results of human infections in December 2019 that it hurts all aspects of life in general and human health in particular. Therefore, it requires addressing such an epidemic quickly and with tight steps to avoid aggravating the situation, especially the lack of appropriate treatment. The necessity necessitated the use of quarantine for the injured and social distancing, in addition to the use of preventive measures such as masks, hand sterilization, non-contact, and leaving a safe distance. This paper aims to use an ANN algorithm based on CT and some laboratory and clinical parameters to determine whether a person is infected with Covid-19 or not. The results showed that two hidden layers were chosen for the ANN algorithm, where the first hidden layer was installed with ten nodes, while the second hidden layer was selected with five nodes once, ten nodes again, fifteen nodes, and twenty nodes. The results showed the best two hidden layers 10-20 nodes, and the accuracy was 99.43%.
Downloads
References
WHO. ((Accessed on 29 April 2022).). World Health Organization. Available: https://www.who.int/emergencies/overview.
C. Huang, Y. Wang, X. Li, L. Ren, J. Zhao, Y. Hu, et al., "Clinical features of patients infected with 2019 novel coronavirus in Wuhan, China," The lancet, vol. 395, pp. 497-506, 2020.
C. Li, C. Zhao, J. Bao, B. Tang, Y. Wang, and B. Gu, "Laboratory diagnosis of coronavirus disease-2019 (COVID-19)," Clinica Chimica Acta, vol. 510, pp. 35-46, 2020.
H. Mahmoud, M. S. Taha, A. Askoura, M. Aleem, A. Omran, and S. Aboelela, "Can chest CT improve sensitivity of COVID-19 diagnosis in comparison to PCR? A meta-analysis study," The Egyptian Journal of Otolaryngology, vol. 36, pp. 1-7, 2020.
C. Jalaber, G. Chassagnon, C. Hani, S. Dangeard, M. Babin, O. Launay, et al., "Is COVID-19 pneumonia differentiable from other viral pneumonia on CT scan?," Respiratory medicine and research, vol. 79, p. 100824, 2021.
S. Altmayer, M. Zanon, G. S. Pacini, G. Watte, M. C. Barros, T.-L. Mohammed, et al., "Comparison of the computed tomography findings in COVID-19 and other viral pneumonia in immunocompetent adults: a systematic review and meta-analysis," European radiology, vol. 30, pp. 6485-6496, 2020.
T. D. Pham, "A comprehensive study on classification of COVID-19 on computed tomography with pretrained convolutional neural networks," Scientific reports, vol. 10, pp. 1-8, 2020.
Z. Yang, Y. Hou, Z. Chen, L. Zhang, and J. Chen, "A Multi-Stage Progressive Learning Strategy for Covid-19 Diagnosis Using Chest Computed Tomography with Imbalanced Data," in ICASSP 2021-2021 IEEE International Conference on Acoustics, Speech, and Signal Processing (ICASSP), 2021, pp. 8578-8582.
P. Dutta, T. Roy, and N. Anjum, "COVID-19 detection using transfer learning with convolutional neural network," in 2021 2nd International Conference on Robotics, Electrical and Signal Processing Techniques (ICREST), 2021, pp. 429-432.
P. Afshar, S. Heidarian, F. Naderkhani, M. J. Rafiee, A. Oikonomou, K. N. Plataniotis, et al., "Hybrid Deep Learning Model For Diagnosis Of Covid-19 Using Ct Scans And Clinical/Demographic Data," in 2021 IEEE International Conference on Image Processing (ICIP), 2021, pp. 180-184.
R. Wang, Z. Jiao, L. Yang, J. W. Choi, Z. Xiong, K. Halsey, et al., "Artificial intelligence for prediction of COVID-19 progression using CT imaging and clinical data," European radiology, pp. 1-8, 2021.
M. Pourhomayoun and M. Shakibi, "Predicting mortality risk in patients with COVID-19 using machine learning to help medical decision-making," Smart Health, vol. 20, p. 100178, 2021.
C. Liu, X. Wang, C. Liu, Q. Sun, and W. Peng, "Differentiating novel coronavirus pneumonia from general pneumonia based on machine learning," Biomedical engineering online, vol. 19, pp. 1-14, 2020.
Z. Yu, X. Li, H. Sun, J. Wang, T. Zhao, H. Chen, et al., "Rapid identification of COVID-19 severity in CT scans through classification of deep features," BioMedical Engineering OnLine, vol. 19, pp. 1-13, 2020.
F. Qeadan, B. Tingey, L. Y. Gu, A. H. Packard, E. Erdei, and A. I. Saeed, "Prognostic values of serum ferritin and D-dimer trajectory in patients with COVID-19," Viruses, vol. 13, p. 419, 2021.
D. Svozil, V. Kvasnicka, and J. Pospichal, "Introduction to multi-layer feed-forward neural networks," Chemometrics and intelligent laboratory systems, vol. 39, pp. 43-62, 1997.
M. A. Khan, R. Khan, F. Algarni, I. Kumar, A. Choudhary, and A. Srivastava, "Performance evaluation of regression models for COVID-19: A statistical and predictive perspective," Ain Shams Engineering Journal, vol. 13, p. 101574, 2022.
L. V. Fausett, Fundamentals of neural networks: architectures, algorithms, and applications: Pearson Education India, 2006.
G. Toğa, B. Atalay, and M. D. Toksari, "COVID-19 prevalence forecasting using autoregressive integrated moving average (ARIMA) and artificial neural networks (ANN): Case of Turkey," Journal of Infection and Public Health, vol. 14, pp. 811-816, 2021.
S. Raheja, S. Kasturia, X. Cheng, and M. Kumar, "Machine learning-based diffusion model for prediction of coronavirus-19 outbreak," Neural Computing and Applications, pp. 1-20, 2021.
S. Ghafouri-Fard, H. Mohammad-Rahimi, P. Motie, M. A. Minabi, M. Taheri, and S. Nateghinia, "Application of machine learning in the prediction of COVID-19 daily new cases: A scoping review," Heliyon, vol. 7, p. e08143, 2021.
Z. Car, S. Baressi Šegota, N. Anđelić, I. Lorencin, and V. Mrzljak, "Modeling the spread of COVID-19 infection using a multilayer perceptron," Computational and mathematical methods in medicine, vol. 2020, 2020.
J. Yang and A. Ahmad, "Comparative Study of Evolutionary Artificial Intelligence Approaches to Predict the Rheological Properties of Fresh Concrete."
T. Rahman, M. E. Chowdhury, A. Khandakar, K. R. Islam, K. F. Islam, Z. B. Mahbub, et al., "Transfer learning with deep convolutional neural network (CNN) for pneumonia detection using chest X-ray," Applied Sciences, vol. 10, p. 3233, 2020.
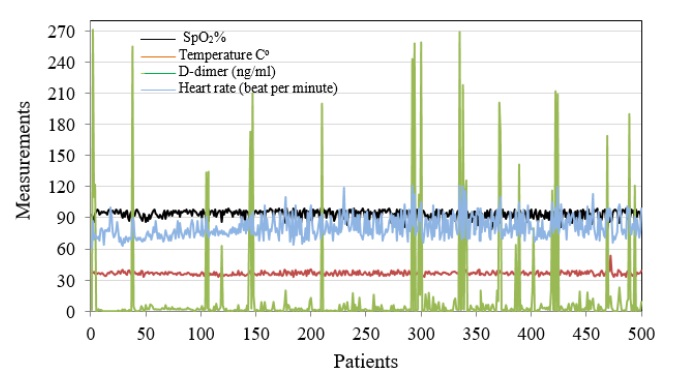
Downloads
Published
How to Cite
Issue
Section
License
Copyright (c) 2022 Humam Adnan Sameer, Ammar Hussein Mutlag, Sadik Kamel Gharghan

This work is licensed under a Creative Commons Attribution 4.0 International License.