Lung Diseases Diagnosis-Based Deep Learning Methods: A Review
DOI:
https://doi.org/10.51173/jt.v5i3.1469Keywords:
COVID-19, CT Scan, Deep Learning, Image Processing, Lung Cancer, Pneumonia, TuberculosisAbstract
This review paper examines the current state of lung disease diagnosis based on deep learning (DL) methods. Lung diseases, such as Pneumonia, TB, Covid-19, and lung cancer, are significant causes of morbidity and mortality worldwide. Accurate and timely diagnosis of these diseases is essential for effective treatment and improved patient outcomes. DL methods, which utilize artificial neural networks to extract features from medical images automatically, have shown great promise in improving the accuracy and efficiency of lung disease diagnosis. This review discusses the various DL methods that have been developed for lung disease diagnosis, including convolutional neural networks (CNNs), deep neural networks (DNNs), and generative adversarial networks (GANs). The advantages and limitations of each method are discussed, along with the types of medical imaging techniques used, such as X-ray and computed tomography (CT). In addition, the review discusses the most commonly used performance metrics for evaluating the performance of DL for lung disease diagnosis: the area under the curve (AUC), sensitivity, specificity, F1-score, accuracy, precision, and the receiver operator characteristic curve (ROC). Moreover, the challenges and limitations of using DL for lung disease diagnosis, including the limited availability of annotated data, the variability in imaging techniques and disease presentation, and the interpretability and generalizability of DL models, are highlighted in this paper. Furthermore, strategies to overcome these challenges, such as transfer learning, data augmentation, and explainable AI, are also discussed. The review concludes with a call for further research to address the remaining challenges and realize DL's full potential for improving lung disease diagnosis and treatment.
Downloads
References
Forum of International Respiratory Societies. The Global Impact of Respiratory Disease, 2nd ed.; European Respiratory Society, Sheffield, UK, 2017; pp. 5–42.
M. M. Rahaman et al., "Identification of COVID-19 samples from chest X-Ray images using deep learning: A comparison of transfer learning approaches," J Xray Sci Technol, vol. 28, no. 5, pp. 821-839, 2020, doi: 10.3233/XST-200715.
R. Loddenkemper, G. Gibson, and Y. Sibille, "The burden of lung disease in Europe: why a European White Book on lung disease?," vol. 22, ed: Eur Respiratory Soc, 2003, pp. 869-69.doi: 10.1183/09031936.03.00107803
O. E. Amani Yahiaoui and N. Yumusak, "A new method of automatic recognition for tuberculosis disease diagnosis using support vector machines," Biomedical Research, vol. 28, no. 9, pp. 4208-4212, 2017.
A. A. A. Setio et al., "Validation, comparison, and combination of algorithms for automatic detection of pulmonary nodules in computed tomography images: The LUNA16 challenge," Med Image Anal, vol. 42, pp. 1-13, Dec 2017, doi: 10.1016/j.media.2017.06.015.
C. Wu, C. Luo, N. Xiong, W. Zhang, and T.-H. Kim, "A Greedy Deep Learning Method for Medical Disease Analysis," IEEE Access, vol. 6, pp. 20021-20030, 2018, doi: 10.1109/access.2018.2823979.
S. Hu et al., "Weakly supervised deep learning for covid-19 infection detection and classification from ct images," IEEE Access, vol. 8, pp. 118869-118883, 2020.doi: 10.1109/ACCESS.2020.3005510.
C. C. Veghes, "The State of Artificial Intelligence in Medical Imaging," 2021.
Y. Zhang, J. M. Gorriz, and Z. Dong, "Deep learning in medical image analysis," vol. 7, ed: MDPI, 2021, p. 74.doi: https://doi.org/10.3390/app11041675
A. A. Mukhlif, B. Al-Khateeb, and M. Mohammed, "Classification of breast cancer images using new transfer learning techniques," Iraqi Journal For Computer Science and Mathematics, vol. 4, no. 1, pp. 167-180, 2023.doi: https://doi.org/10.52866/ijcsm.2023.01.01.0014
A. S. Lundervold and A. Lundervold, "An overview of deep learning in medical imaging focusing on MRI," Zeitschrift für Medizinische Physik, vol. 29, no. 2, pp. 102-127, 2019.doi: https://doi.org/10.1016/j.zemedi.2018.11.002
L. Alzubaidi et al., "Novel transfer learning approach for medical imaging with limited labeled data," Cancers, vol. 13, no. 7, p. 1590, 2021.doi: https://doi.org/10.3390/cancers13071590
A. N. Zghair, "Assessment of Haematological Parameters in Drug-Resistant TB," Journal of Techniques, vol. 5, no. 1, pp. 137-143, 2023.doi: , doi: https://doi.org/10.51173/jt.v5i1.1082
P. Van Dyck, F. Vanhoenacker, P. Van den Brande, and A. De Schepper, "Imaging of pulmonary tuberculosis," European radiology, vol. 13, pp. 1771-1785, 2003. doi:https://doi.org/10.1007/s00330-002-1612-y
A. Khatri, R. Jain, H. Vashista, N. Mittal, P. Ranjan, and R. Janardhanan, "Pneumonia identification in chest X-ray images using EMD," in Trends in Communication, Cloud, and Big Data: Proceedings of 3rd National Conference on CCB, 2018, 2020: Springer, pp. 87-98. doi: 10.1007/978-981-15-1624-5_9
E. Ayan and H. M. Ünver, "Diagnosis of pneumonia from chest X-ray images using deep learning," in 2019 Scientific Meeting on Electrical-Electronics & Biomedical Engineering and Computer Science (EBBT), 2019: Ieee, pp. 1-5. doi: 10.1109/EBBT.2019.8741582
A. C. Borczuk, "Benign tumors and tumorlike conditions of the lung," Archives of pathology & laboratory medicine, vol. 132, no. 7, pp. 1133-1148, 2008.doi: https://doi.org/10.3390/jimaging6120131
Q. Song, L. Zhao, X. Luo, and X. Dou, "Using deep learning for classification of lung nodules on computed tomography images," Journal of healthcare engineering, vol. 2017, 2017.doi: https://doi.org/10.1155/2017/8314740
A. Webb, Introduction to biomedical imaging. John Wiley & Sons, 2022.
K.-H. Ng and M. M. Rehani, "X ray imaging goes digital," vol. 333, ed: British Medical Journal Publishing Group, 2006, pp. 765-766.doi: 10.1136/bmj.38977.669769.2C
D. S. Kermany et al., "Identifying medical diagnoses and treatable diseases by image-based deep learning," cell, vol. 172, no. 5, pp. 1122-1131. e9, 2018.doi: https://doi.org/10.1016/j.cell.2018.02.010
M. G. F. Costa, C. F. Costa Filho, A. Kimura, P. C. Levy, C. M. Xavier, and L. Fujimoto, "A sputum smear microscopy image database for automatic bacilli detection in conventional microscopy," in 2014 36th Annual International Conference of the IEEE Engineering in Medicine and Biology Society, 2014: IEEE, pp. 2841-2844. doi: 10.1109/EMBC.2014.6944215
X. Wang, Y. Peng, L. Lu, Z. Lu, M. Bagheri, and R. M. Summers, "Chestx-ray8: Hospital-scale chest x-ray database and benchmarks on weakly-supervised classification and localization of common thorax diseases," in Proceedings of the IEEE conference on computer vision and pattern recognition, 2017, pp. 2097-2106. doi: https://www.cc.nih.gov/drd/summers.html
M. E. Chowdhury et al., "Can AI help in screening viral and COVID-19 pneumonia?," Ieee Access, vol. 8, pp. 132665-132676, 2020.doi: 10.1109/ACCESS.2020.3010287
G. T. Herman, Fundamentals of computerized tomography: image reconstruction from projections. Springer Science & Business Media, 2009.doi: https://link.springer.com/book/10.1007/978-1-84628-723-7
J. Shiraishi et al., "Development of a digital image database for chest radiographs with and without a lung nodule: receiver operating characteristic analysis of radiologists' detection of pulmonary nodules," American Journal of Roentgenology, vol. 174, no. 1, pp. 71-74, 2000.doi: 10.2214/ajr.174.1.1740071
Y. D. Cid, V. Liauchuk, D. Klimuk, A. Tarasau, V. Kovalev, and H. Müller, "Overview of ImageCLEFtuberculosis 2019-Automatic CT-based Report Generation and Tuberculosis Severity Assessment," in CLEF (Working Notes), 2019.
S. Diciotti, S. Lombardo, M. Falchini, G. Picozzi, and M. Mascalchi, "Automated segmentation refinement of small lung nodules in CT scans by local shape analysis," IEEE Transactions on Biomedical Engineering, vol. 58, no. 12, pp. 3418-3428, 2011.doi: 10.1109/TBME.2011.2167621.
J. P. Cohen, P. Morrison, L. Dao, K. Roth, T. Q. Duong, and M. Ghassemi, "Covid-19 image data collection: Prospective predictions are the future," arXiv preprint arXiv:2006.11988, 2020.doi: https://doi.org/10.48550/arXiv.2006.11988.
E. Al Hadhrami, M. Al Mufti, B. Taha, and N. Werghi, "Transfer learning with convolutional neural networks for moving target classification with micro-Doppler radar spectrograms," in 2018 International Conference on Artificial Intelligence and Big Data (ICAIBD), 2018: IEEE, pp. 148-154. doi: 10.1109/ICAIBD.2018.8396184
O. Sevli, "A deep convolutional neural network-based pigmented skin lesion classification application and experts evaluation," Neural Computing and Applications, vol. 33, no. 18, pp. 12039-12050, 2021.doi: https://doi.org/10.1007/s00521-021-05929-4
K. Simonyan and A. Zisserman, "Very deep convolutional networks for large-scale image recognition," arXiv preprint arXiv:1409.1556, 2014.doi: https://doi.org/10.48550/arXiv.1409.1556.
H. Janoria, J. Minj, and P. Patre, "Classification of skin disease from skin images using transfer learning technique," in 2020 4th International Conference on Electronics, Communication and Aerospace Technology (ICECA), 2020: IEEE, pp. 888-895. doi: 10.1109/ICECA49313.2020.9297567.
H. Qassim, A. Verma, and D. Feinzimer, "Compressed residual-VGG16 CNN model for big data places image recognition," in 2018 IEEE 8th annual computing and communication workshop and conference (CCWC), 2018: IEEE, pp. 169-175. doi: 10.1109/CCWC.2018.8301729.
F. Chollet, "Xception: Deep learning with depthwise separable convolutions," in Proceedings of the IEEE conference on computer vision and pattern recognition, 2017, pp. 1251-1258. doi: 10.1109/CVPR.2017.195.
M. M. A. Shibly, T. A. Tisha, T. A. Tani, and S. Ripon, "Convolutional neural network-based ensemble methods to recognize Bangla handwritten character," PeerJ Computer Science, vol. 7, p. e565, 2021.doi: https://doi.org/10.7717/peerj-cs.565.
P. Gifani, A. Shalbaf, and M. Vafaeezadeh, "Automated detection of COVID-19 using ensemble of transfer learning with deep convolutional neural network based on CT scans," International journal of computer assisted radiology and surgery, vol. 16, pp. 115-123, 2021.doi: https://doi.org/10.1007/s11548-020-02286-w.
Y. Zou, L. Zhao, S. Qin, M. Pan, and Z. Li, "Ship target detection and identification based on SSD_MobilenetV2," in 2020 IEEE 5th Information Technology and Mechatronics Engineering Conference (ITOEC), 2020: IEEE, pp. 1676-1680. doi: 10.1109/ITOEC49072.2020.9141734.
M. Sandler, A. Howard, M. Zhu, A. Zhmoginov, and L.-C. Chen, "Mobilenetv2: Inverted residuals and linear bottlenecks," in Proceedings of the IEEE conference on computer vision and pattern recognition, 2018, pp. 4510-4520.:https://openaccess.thecvf.com/content_cvpr_2018/papers/Sandler_MobileNetV2_Inverted_Residuals_CVPR_2018_paper.pdf.
A. V. Ikechukwu, S. Murali, R. Deepu, and R. Shivamurthy, "ResNet-50 vs VGG-19 vs training from scratch: A comparative analysis of the segmentation and classification of Pneumonia from chest X-ray images," Global Transitions Proceedings, vol. 2, no. 2, pp. 375-381, 2021.doi: https://doi.org/10.1016/j.gltp.2021.08.027.
S. Rajaraman et al., "A novel stacked generalization of models for improved TB detection in chest radiographs," in 2018 40th Annual International Conference of the IEEE Engineering in Medicine and Biology Society (EMBC), 2018: IEEE, pp. 718-721. doi: https://doi.org/10.1007/s00521-021-06177-2.
G. Capizzi, G. L. Sciuto, C. Napoli, D. Połap, and M. Woźniak, "Small lung nodules detection based on fuzzy-logic and probabilistic neural network with bioinspired reinforcement learning," IEEE Transactions on Fuzzy Systems, vol. 28, no. 6, pp. 1178-1189, 2019.doi: 10.1109/TFUZZ.2019.2952831.
T. K. K. Ho and J. Gwak, "Utilizing Knowledge Distillation in Deep Learning for Classification of Chest X-Ray Abnormalities," IEEE Access, vol. 8, pp. 160749-160761, 2020, doi: 10.1109/access.2020.3020802.
R. R. N. Tobias et al., "CNN-based deep learning model for chest X-ray health classification using tensorflow," in 2020 RIVF International Conference on Computing and Communication Technologies (RIVF), 2020: IEEE, pp. 1-6. doi: 10.1109/RIVF48685.2020.9140733.
S. Gite, A. Mishra, and K. Kotecha, "Enhanced lung image segmentation using deep learning," Neural Comput Appl, pp. 1-15, Jan 3 2022, doi: 10.1007/s00521-021-06719-8.
Y. H. Bhosale and K. Sridhar Patnaik, "IoT Deployable Lightweight Deep Learning Application For COVID-19 Detection With Lung Diseases Using RaspberryPi," presented at the 2022 International Conference on IoT and Blockchain Technology (ICIBT), 2022.doi: 10.1109/ICIBT52874.2022.9807725.
A. Khadidos, A. O. Khadidos, S. Kannan, Y. Natarajan, S. N. Mohanty, and G. Tsaramirsis, "Analysis of covid-19 infections on a ct image using deepsense model," Frontiers in public health, vol. 8, p. 599550, 2020.doi: https://doi.org/10.3389/fpubh.2020.599550.
Z. Wang, H. Xu, and M. Sun, "Deep learning based nodule detection from pulmonary CT images," in 2017 10th International Symposium on Computational Intelligence and Design (ISCID), 2017, vol. 1: IEEE, pp. 370-373. doi: 10.1109/ISCID.2017.107.
P. Yadav, N. Menon, V. Ravi, and S. Vishvanathan, "Lung-gans: Unsupervised representation learning for lung disease classification using chest ct and x-ray images," IEEE Transactions on Engineering Management, 2021.doi: 10.1109/TEM.2021.3103334,
H. Tang, C. Zhang, and X. Xie, "Automatic pulmonary lobe segmentation using deep learning," in 2019 IEEE 16th international symposium on biomedical imaging (ISBI 2019), 2019: IEEE, pp. 1225-1228. doi: 10.1109/ISBI.2019.8759468.
H. Hou, W. Lv, and Q. Tao, "Hospital, T., Company, JT, Ai, T," Hospital, T., Wuhan, T., & Hospital, 2020.
A. A. Ardakani, A. R. Kanafi, U. R. Acharya, N. Khadem, and A. Mohammadi, "Application of deep learning technique to manage COVID-19 in routine clinical practice using CT images: Results of 10 convolutional neural networks," Computers in biology and medicine, vol. 121, p. 103795, 2020.doi: https://doi.org/10.1016/j.compbiomed.2020.103795.
A. M. Hasan, M. M. Al-Jawad, H. A. Jalab, H. Shaiba, R. W. Ibrahim, and A. a. R. AL-Shamasneh, "Classification of Covid-19 coronavirus, pneumonia and healthy lungs in CT scans using Q-deformed entropy and deep learning features," Entropy, vol. 22, no. 5, p. 517, 2020.doi: https://doi.org/10.3390/e22050517.
Y. Pathak, P. K. Shukla, A. Tiwari, S. Stalin, and S. Singh, "Deep transfer learning based classification model for COVID-19 disease," Irbm, 2020.doi: 10.1016/j.irbm.2020.05.003.
T. Yan, P. K. Wong, H. Ren, H. Wang, J. Wang, and Y. Li, "Automatic distinction between COVID-19 and common pneumonia using multi-scale convolutional neural network on chest CT scans," Chaos, Solitons & Fractals, vol. 140, p. 110153, 2020.doi: 10.1016/j.chaos.2020.110153.
C. Liu, X. Wang, C. Liu, Q. Sun, and W. Peng, "Differentiating novel coronavirus pneumonia from general pneumonia based on machine learning," Biomedical engineering online, vol. 19, no. 1, pp. 1-14, 2020.doi: https://doi.org/10.1186/s12938-020-00809-9.
L. Li et al., "Artificial intelligence distinguishes COVID-19 from community acquired pneumonia on chest CT. Radiology," ed, 2020.doi: https://doi.org/10.1038/s41467-020-17971-2.
S. Wang et al., "A deep learning algorithm using CT images to screen for Corona Virus Disease (COVID-19)," European radiology, vol. 31, no. 8, pp. 6096-6104, 2021. 10.1007/s00330-021-07715-1.
T. A. U.-M. Sadoon and M. H. Ali, "Coronavirus 2019 (COVID-19) Detection Based on Deep Learning," Al-Nahrain Journal for Engineering Sciences, vol. 23, no. 4, pp. 408-415, 2020.doi: 10.1007/s42979-022-01464-8.
M. Barstugan, U. Ozkaya, and S. Ozturk, "Coronavirus (covid-19) classification using ct images by machine learning methods," arXiv preprint arXiv:2003.09424, 2020.doi: https://doi.org/10.48550/arXiv.2003.09424.
V. Shah, R. Keniya, A. Shridharani, M. Punjabi, J. Shah, and N. Mehendale, "Diagnosis of COVID-19 using CT scan images and deep learning techniques," Emergency radiology, vol. 28, no. 3, pp. 497-505, 2021.doi: https://doi.org/10.1007/s10140-020-01886-y.
H. Panwar, P. Gupta, M. K. Siddiqui, R. Morales-Menendez, P. Bhardwaj, and V. Singh, "A deep learning and grad-CAM based color visualization approach for fast detection of COVID-19 cases using chest X-ray and CT-Scan images," Chaos, Solitons & Fractals, vol. 140, p. 110190, 2020.doi: 10.1016/j.chaos.2020.110190.
L. N. Rohmah and A. Bustamam, "Improved classification of coronavirus disease (COVID-19) based on combination of texture features using CT scan and X-ray images," in 2020 3rd International Conference on Information and Communications Technology (ICOIACT), 2020: IEEE, pp. 105-109. doi: covidwho-1105152.
X. Wang et al., "A weakly-supervised framework for COVID-19 classification and lesion localization from chest CT," IEEE transactions on medical imaging, vol. 39, no. 8, pp. 2615-2625, 2020.doi: 10.1016/j.bspc.2022.104099.
M. Fraiwan, L. Fraiwan, M. Alkhodari, and O. Hassanin, "Recognition of pulmonary diseases from lung sounds using convolutional neural networks and long short-term memory," Journal of Ambient Intelligence and Humanized Computing, pp. 1-13, 2021.doi: https://doi.org/10.1007/s12652-021-03184-y.
L. Pham, H. Phan, R. Palaniappan, A. Mertins, and I. McLoughlin, "CNN-MoE Based Framework for Classification of Respiratory Anomalies and Lung Disease Detection," IEEE J Biomed Health Inform, vol. 25, no. 8, pp. 2938-2947, Aug 2021, doi: 10.1109/JBHI.2021.3064237.
M. Aykanat, Ö. Kılıç, B. Kurt, and S. Saryal, "Classification of lung sounds using convolutional neural networks," EURASIP Journal on Image and Video Processing, vol. 2017, no. 1, p. 65, 2017/09/11 2017, doi: 10.1186/s13640-017-0213-2.
R. Hazra and S. Majhi, "Detecting respiratory diseases from recorded lung sounds by 2d cnn," in 2020 5th International Conference on Computing, Communication and Security (ICCCS), 2020: IEEE, pp. 1-6. doi: 10.1109/ICCCS49678.2020.9277101.
S. Kumar et al., "Chest X ray and cough sample based deep learning framework for accurate diagnosis of COVID-19," Computers and Electrical Engineering, vol. 103, p. 108391, 2022.doi: https://doi.org/10.1016/j.compeleceng.2022.108391.
M. Fraiwan, L. Fraiwan, M. Alkhodari, and O. Hassanin, "Recognition of pulmonary diseases from lung sounds using convolutional neural networks and long short-term memory," J Ambient Intell Humaniz Comput, vol. 13, no. 10, pp. 4759-4771, 2022, doi: 10.1007/s12652-021-03184-y.
S. V. Stehman, "Selecting and interpreting measures of thematic classification accuracy," Remote sensing of Environment, vol. 62, no. 1, pp. 77-89, 1997.doi: https://doi.org/10.1016/S0034-4257(97)00083-7.
A. A. Mukhlif, B. Al-Khateeb, and M. A. Mohammed, "An extensive review of state-of-the-art transfer learning techniques used in medical imaging: Open issues and challenges," Journal of Intelligent Systems, vol. 31, no. 1, pp. 1085-1111, 2022.doi: https://doi.org/10.1515/jisys-2022-0198.
S. Wu, W.-C. Yau, T.-S. Ong, and S.-C. Chong, "Integrated churn prediction and customer segmentation framework for telco business," IEEE Access, vol. 9, pp. 62118-62136, 2021.doi: 10.1109/ACCESS.2021.3073776.
B. Savelli, A. Bria, M. Molinara, C. Marrocco, and F. Tortorella, "A multi-context CNN ensemble for small lesion detection," Artificial Intelligence in Medicine, vol. 103, p. 101749, 2020.doi: https://doi.org/10.1016/j.artmed.2019.101749.
A. Al-Ajlan and A. El Allali, "CNN-MGP: convolutional neural networks for metagenomics gene prediction," Interdisciplinary Sciences: Computational Life Sciences, vol. 11, pp. 628-635, 2019.doi: https://doi.org/10.1007/s12539-018-0313-4.
G. An, M. Akiba, K. Omodaka, T. Nakazawa, and H. Yokota, "Hierarchical deep learning models using transfer learning for disease detection and classification based on small number of medical images," Scientific reports, vol. 11, no. 1, p. 4250, 2021.doi: https://doi.org/10.1038/s41598-021-83503-7.
V. Taran, Y. Gordienko, O. Rokovyi, O. Alienin, Y. Kochura, and S. Stirenko, "Edge intelligence for medical applications under field conditions," in Advances in Artificial Systems for Logistics Engineering: Springer, 2022, pp. 71-80.doi: https://doi.org/10.1007/978-3-031-04809-8_6.
K. A. Tran, O. Kondrashova, A. Bradley, E. D. Williams, J. V. Pearson, and N. Waddell, "Deep learning in cancer diagnosis, prognosis and treatment selection," Genome Medicine, vol. 13, no. 1, pp. 1-17, 2021.doi: https://doi.org/10.1186/s13073-021-00968-x.
S. T. Ahmed and S. M. Kadhem, "Using Machine Learning via Deep Learning Algorithms to Diagnose the Lung Disease Based on Chest Imaging: A Survey," International Journal of Interactive Mobile Technologies, vol. 15, no. 16, 2021.doi: 10.3991/ijim.v15i16.24191.
Q. Guan et al., "Medical image augmentation for lesion detection using a texture-constrained multichannel progressive GAN," Computers in Biology and Medicine, vol. 145, p. 105444, 2022.doi: https://doi.org/10.1016/j.compbiomed.2022.105444.
F. Munawar, S. Azmat, T. Iqbal, C. Grönlund, and H. Ali, "Segmentation of lungs in chest X-ray image using generative adversarial networks," Ieee Access, vol. 8, pp. 153535-153545, 2020.doi: 10.1016/j.compbiomed.2022.105444
M. Kim et al., "Deep learning in medical imaging," Neurospine, vol. 16, no. 4, p. 657, 2019.
Y. Li and X. Chao, "Toward sustainability: trade-off between data quality and quantity in crop pest recognition," Frontiers in plant science, vol. 12, p. 2959, 2021.doi: 10.3389/fpls.2021.811241.
T. Han, Y.-F. Li, and M. Qian, "A hybrid generalization network for intelligent fault diagnosis of rotating machinery under unseen working conditions," IEEE Transactions on Instrumentation and Measurement, vol. 70, pp. 1-11, 2021.doi: https://doi.org/10.1007/s11465-021-0652-4.
R. ElShawi, Y. Sherif, M. Al‐Mallah, and S. Sakr, "Interpretability in healthcare: A comparative study of local machine learning interpretability techniques," Computational Intelligence, vol. 37, no. 4, pp. 1633-1650, 2021.doi: 10.1111/coin.12410.
F. Renard, S. Guedria, N. D. Palma, and N. Vuillerme, "Variability and reproducibility in deep learning for medical image segmentation," Scientific Reports, vol. 10, no. 1, pp. 1-16, 2020.doi: https://doi.org/10.1038/s41598-020-69920-0.
L. Gao, L. Zhang, C. Liu, and S. Wu, "Handling imbalanced medical image data: A deep-learning-based one-class classification approach," Artificial intelligence in medicine, vol. 108, p. 101935, 2020.doi: https://doi.org/10.1016/j.artmed.2020.101935.
Y. Bengio, A. Lodi, and A. Prouvost, "Machine learning for combinatorial optimization: a methodological tour d’horizon," European Journal of Operational Research, vol. 290, no. 2, pp. 405-421, 2021.doi: https://doi.org/10.1016/j.ejor.2020.07.063.
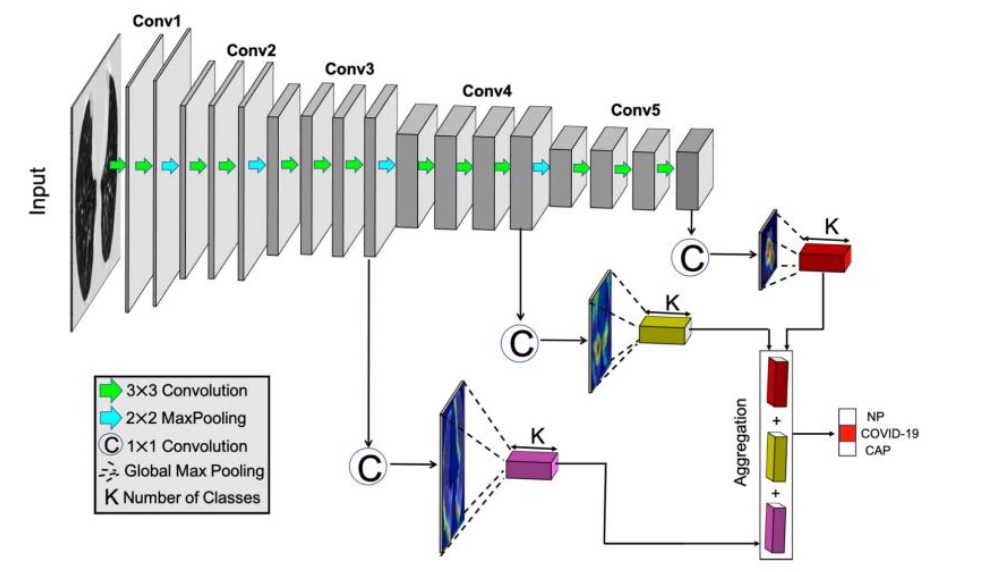
Downloads
Published
How to Cite
Issue
Section
License
Copyright (c) 2023 Shahad A. Salih, Sadik Kamel Gharghan, Jinan F. Mahdi, Inas Jawad Kadhim

This work is licensed under a Creative Commons Attribution 4.0 International License.