A Decision Cloud Ranking Approach Based on Privacy and Security in Blockchain E-Health Industry 4.0 Systems
DOI:
https://doi.org/10.51173/jt.v5i4.1464Keywords:
E-Health Systems, Industry 4.0, Multi-Criteria Decision-Making, Security, PrivacyAbstract
E-health Industry 4.0 systems ranking based on Blockchain is a multi-criteria decision-making (MCDM) problem, considering the multiple evaluation properties, their significance, and data variety. The final closeness between the evaluation sample and ideal solutions also constitutes an optimisation problem. To the authors’ knowledge, no study has provided a multi-privacy and security ranking approach solution for E-health Industry 4.0 systems based on Blockchain. Consequently, this study proposes and discusses a multi-privacy and security ranking approach solution for E-health Industry 4.0 systems, utilising the SFS-FWZIC method to address the significance of properties issue and the Grey-TOPSIS method to tackle data variation, multiple evaluation properties, and the ideal solutions optimisation problem. The methodology of the proposed approach consists of three sections. First, three decision matrices are constructed based on the intersection of E-health systems with the mentioned properties, intersecting Electronic Health Records (EHRs), Electronic Medical Records (EMRs), and Personal Health Records (PHRs) with seven properties. Second, the weights of each privacy and security property are calculated using the SFS-FWZIC method. Finally, the weights determined by the SFS-FWZIC method and the three decision matrices are input into the Grey-TOPSIS method to rank E-health systems across the three categories. The findings reveal the following: (1) The SFS-FWZIC method effectively assigned weights to privacy and security properties, with access control achieving the highest significance weight (0.1934) and Secure-search receiving the lowest weight (0.0603). (2) The Grey-TOPSIS method efficiently ranked E-health systems across the three categories based on various parameters, including α=0.1,α=0.3,α=0.5,α=0.7, and α=0.9. Sensitivity and correlation analysis were conducted to evaluate the results, revealing high correlation results based on each α value across all discussed scenarios of changing property weights. The implications of this study can lead to better decision-making, improved security and privacy, increased competition, and widespread adoption, ultimately contributing to more efficient and effective healthcare delivery.
Downloads
References
G. Seaman, “Industrial/management engineering in health care,” in Clinical Engineering Handbook, Elsevier Science Ltd., 2004, pp. 181–188. doi: 10.1016/B978-012226570-9/50052-1.
T. Materla, E. A. Cudney, and J. Antony, “The application of Kano model in the healthcare industry: a systematic literature review,” Total Quality Management and Business Excellence, vol. 30, no. 5–6. Routledge, pp. 660–681, Apr. 03, 2019. doi: 10.1080/14783363.2017.1328980.
M. Chatfield, “Health United States,” in Health Care Management Review, 1979, p. 86. doi: 10.1097/00004010-197900440-00013.
S. P. Keehan et al., “National health expenditure projections, 2019–28: Expected rebound in prices drives rising spending growth,” Health Aff, vol. 39, no. 4, pp. 704–714, Apr. 2020, doi: 10.1377/hlthaff.2020.00094.
G. Aceto, V. Persico, and A. Pescapé, “Industry 4.0 and Health: Internet of Things, Big Data, and Cloud Computing for Healthcare 4.0,” J Ind Inf Integr, vol. 18, 2020, doi: 10.1016/j.jii.2020.100129.
M. Kumar and S. Chand, “A lightweight cloud-assisted identity-based anonymous authentication and key agreement protocol for secure wireless body area network,” IEEE Syst J, vol. 15, no. 2, pp. 2779–2786, 2021, doi: 10.1109/JSYST.2020.2990749.
K. A. Mkalaf and P. Gibson, “Application the Risk-Based Maintenance for Optimising the Overall Medical Devices Safety,” Journal of Techniques, vol. 4, no. 4, pp. 111–118, Dec. 2022, doi: 10.51173/JT.V4I4.615.
H. Khalid Malik, J. Al-Anber, F. Abdo, and E. Al-Mekhlafi, “Comparison of Feature Selection and Feature Extraction Role in Dimensionality Reduction of Big Data,” Journal of Techniques, vol. 5, no. 1, pp. 184–192, Apr. 2023, doi: 10.51173/JT.V5I1.1027.
M. Kumar and S. Chand, “MedHypChain: A patient-centred interoperability hyperledger-based medical healthcare system: Regulation in COVID-19 pandemic,” Journal of Network and Computer Applications, vol. 179, 2021, doi: 10.1016/j.jnca.2021.102975.
K. Abouelmehdi, A. Beni-Hessane, and H. Khaloufi, “Big healthcare data: preserving security and privacy,” J Big Data, vol. 5, no. 1, Dec. 2018, doi: 10.1186/s40537-017-0110-7.
G. Zhao et al., “Blockchain technology in agri-food value chain management: A synthesis of applications, challenges and future research directions,” Comput Ind, vol. 109, pp. 83–99, 2019, doi: 10.1016/j.compind.2019.04.002.
H. F. Jawad, A. Al-Askery, and A. H. Ali, “Design and Implementation of a Healthcare Monitoring System Based on LoRa,” Journal of Techniques, vol. 4, no. 4, pp. 80–94, Dec. 2022, doi: 10.51173/JT.V4I4.792.
R. N. Hussein, G. Nassreddine, and J. Younis, “The Impact of Information Technology Integration on the Decision-Making Process,” Journal of Techniques, vol. 5, no. 1, pp. 144–155, Apr. 2023, doi: 10.51173/JT.V5I1.1262.
S. Tanwar, K. Parekh, and R. Evans, “Blockchain-based electronic healthcare record system for healthcare 4.0 applications,” Journal of Information Security and Applications, vol. 50, p. 102407, 2020, doi: 10.1016/j.jisa.2019.102407.
A. Khatoon, “A blockchain-based smart contract system for healthcare management,” Electronics (Switzerland), vol. 9, no. 1, p. 94, Jan. 2020, doi: 10.3390/electronics9010094.
H. M. Hussien, S. M. Yasin, N. I. Udzir, M. I. H. Ninggal, and S. Salman, “Blockchain technology in the healthcare industry: Trends and opportunities,” J Ind Inf Integr, vol. 22, 2021, doi: 10.1016/j.jii.2021.100217.
S. Qahtan, K. Yatim, H. Zulzalil, M. H. Osman, A. A. Zaidan, and H. A. Alsattar, “Review of healthcare industry 4.0 application-based blockchain in terms of security and privacy development attributes: Comprehensive taxonomy, open issues and challenges and recommended solution,” Journal of Network and Computer Applications, vol. 209, p. 103529, Oct. 2022, doi: 10.1016/J.JNCA.2022.103529.
S. Qahtan et al., “Novel Multi Security and Privacy Benchmarking Framework for Blockchain-Based IoT Healthcare Industry 4.0 Systems,” IEEE Trans Industr Inform, vol. 18, no. 9, pp. 6415–6423, 2022, doi: 10.1109/TII.2022.3143619.
S. Qahtan, A. Alaa Zaidan, H. Abdulsattar Ibrahim, M. Deveci, W. Ding, and D. Pamucar, “A decision modeling approach for smart training environment with motor Imagery-based brain computer interface under neutrosophic cubic fuzzy set,” Expert Syst Appl, vol. 224, p. 119991, Aug. 2023, doi: 10.1016/J.ESWA.2023.119991.
Z. K. Mohammed et al., “Bitcoin network-based anonymity and privacy model for metaverse implementation in Industry 5.0 using linear Diophantine fuzzy sets,” Ann Oper Res, Jun. 2023, doi: 10.1007/s10479-023-05421-3.
A. A. Zaidan, H. A. Alsattar, S. Qahtan, M. Deveci, D. Pamucar, and M. Hajiaghaei-Keshteli, “Uncertainty Decision Modeling Approach for Control Engineering Tools to Support Industrial Cyber-Physical Metaverse Smart Manufacturing Systems,” IEEE Syst J, pp. 1–12, 2023, doi: 10.1109/JSYST.2023.3266842.
M. J. Baqer, H. A. AlSattar, S. Qahtan, A. A. Zaidan, M. A. M. Izhar, and I. T. Abbas, “A Decision Modeling Approach for Data Acquisition Systems of the Vehicle Industry Based on Interval-Valued Linear Diophantine Fuzzy Set,” Int J Inf Technol Decis Mak, Apr. 2023, doi: 10.1142/S0219622023500487.
A. AlSereidi et al., “Novel Federated Decision Making for Distribution of Anti-SARS-CoV-2 Monoclonal Antibody to Eligible High-Risk Patients,” Int J Inf Technol Decis Mak, Aug. 2022, doi: 10.1142/S021962202250050X.
A. S. Albahri et al., “Towards physician’s experience: Development of machine learning model for the diagnosis of autism spectrum disorders based on complex T-spherical fuzzy-weighted zero-inconsistency method,” Comput Intell, 2022, doi: 10.1111/coin.12562.
M. A. Alsalem et al., “Multiclass Benchmarking Framework for Automated Acute Leukaemia Detection and Classification Based on BWM and Group-VIKOR,” J Med Syst, vol. 43, no. 7, pp. 1–32, Jul. 2019, doi: 10.1007/s10916-019-1338-x.
H. A. Alsattar et al., “Integration of FDOSM and FWZIC Under Homogeneous Fermatean Fuzzy Environment: A Prioritisation of COVID-19 Patients for Mesenchymal Stem Cell Transfusion,” Int J Inf Technol Decis Mak, 2022, doi: 10.1142/S0219622022500511.
S. Qahtan, H. A. Alsattar, A. A. Zaidan, M. Deveci, D. Pamucar, and D. Delen, “Performance assessment of sustainable transportation in the shipping industry using a q-rung ortho-pair fuzzy rough sets-based decision making methodology,” Expert Syst Appl, p. 119958, Mar. 2023, doi: 10.1016/J.ESWA.2023.119958.
E. M. Almahdi, A. A. Zaidan, B. B. Zaidan, M. A. Alsalem, O. S. Albahri, and A. S. Albahri, “Mobile Patient Monitoring Systems from a Benchmarking Aspect: Challenges, Open Issues and Recommended Solutions,” J Med Syst, vol. 43, no. 7, pp. 1–23, May 2019, doi: 10.1007/s10916-019-1336-z.
H. A. Ibrahim, A. A. Zaidan, S. Qahtan, and B. B. Zaidan, “Sustainability assessment of palm oil industry 4.0 technologies in a circular economy applications based on interval-valued Pythagorean fuzzy rough set-FWZIC and EDAS methods,” Appl Soft Comput, p. 110073, Feb. 2023, doi: 10.1016/J.ASOC.2023.110073.
U. S. Mahmoud et al., “DAS benchmarking methodology based on FWZIC II and FDOSM II to support industrial community characteristics in the design and implementation of advanced driver assistance systems in vehicles,” J Ambient Intell Humaniz Comput, 2022, doi: 10.1007/s12652-022-04201-4.
A. S. Albahri et al., “Towards physician’s experience: Development of machine learning model for the diagnosis of autism spectrum disorders based on complex T-spherical fuzzy-weighted zero-inconsistency method,” Comput Intell, 2022, doi: 10.1111/coin.12562.
A. A. Zaidan, B. B. Zaidan, M. A. Alsalem, O. S. Albahri, A. S. Albahri, and M. Y. Qahtan, “Multi-agent learning neural network and Bayesian model for real-time IoT skin detectors: a new evaluation and benchmarking methodology,” Neural Comput Appl, vol. 32, no. 12, pp. 8315–8366, Jun. 2020, doi: 10.1007/s00521-019-04325-3.
S. Qahtan, H. A. Alsattar, A. A. Zaidan, M. Deveci, D. Pamucar, and W. Ding, “A novel fuel supply system modelling approach for electric vehicles under Pythagorean probabilistic hesitant fuzzy sets,” Inf Sci (N Y), vol. 622, pp. 1014–1032, Apr. 2023, doi: 10.1016/j.ins.2022.11.166.
A. Alamleh et al., “Multi-Attribute Decision-Making for Intrusion Detection Systems: A Systematic Review,” Int J Inf Technol Decis Mak, Jul. 2022, doi: 10.1142/S021962202230004X.
S. Qahtan, H. A. Alsattar, A. A. Zaidan, M. Deveci, D. Pamucar, and L. Martinez, “A comparative study of evaluating and benchmarking sign language recognition system-based wearable sensory devices using a single fuzzy set,” Knowl Based Syst, p. 110519, Mar. 2023, doi: 10.1016/J.KNOSYS.2023.110519.
F. M. Jumaah, A. A. Zaidan, B. B. Zaidan, R. Bahbibi, M. Y. Qahtan, and A. Sali, “Technique for order performance by similarity to ideal solution for solving complex situations in multi-criteria optimisation of the tracking channels of GPS baseband telecommunication receivers,” Telecommun Syst, vol. 68, no. 3, pp. 425–443, Jul. 2018, doi: 10.1007/s11235-017-0401-5.
Q. M. Yas, A. A. Zaidan, B. B. Zaidan, B. Rahmatullah, and H. Abdul Karim, “Comprehensive insights into evaluation and benchmarking of real-time skin detectors: Review, open issues & challenges, and recommended solutions,” Measurement (Lond), vol. 114, pp. 243–260, 2018, doi: 10.1016/j.measurement.2017.09.027.
S. Qahtan et al., “Evaluation of agriculture-food 4.0 supply chain approaches using Fermatean probabilistic hesitant-fuzzy sets based decision-making model,” Appl Soft Comput, p. 110170, Mar. 2023, doi: 10.1016/J.ASOC.2023.110170.
A. Alamleh et al., “Federated Learning for IoMT Applications: A Standardisation and Benchmarking Framework of Intrusion Detection Systems,” IEEE J Biomed Health Inform, pp. 1–1, 2022, doi: 10.1109/JBHI.2022.3167256.
B. Wu, M. Lu, W. Huang, Y. Lan, Y. Wu, and Z. Huang, “A Case Study on the Construction Optimization Decision Scheme of Urban Subway Tunnel Based on the TOPSIS Method,” KSCE Journal of Civil Engineering, vol. 24, no. 11, pp. 3488–3500, Nov. 2020, doi: 10.1007/s12205-020-1290-9.
X. Yu, X. Wu, and T. Huo, “Combine MCDM methods and PSO to evaluate economic benefits of high-tech zones in China,” Sustainability (Switzerland), vol. 12, no. 18, Sep. 2020, doi: 10.3390/SU12187833.
T. Yang, Q. Zhang, X. Wan, X. Li, Y. Wang, and W. Wang, “Comprehensive ecological risk assessment for semi-arid basin based on conceptual model of risk response and improved TOPSIS model-a case study of Wei River Basin, China,” Science of the Total Environment, vol. 719, p. 137502, 2020, doi: 10.1016/j.scitotenv.2020.137502.
Z. Ding, Z. Jiang, H. Zhang, W. Cai, and Y. Liu, “An integrated decision-making method for selecting machine tool guideways considering manufacturability,” Int J Comput Integr Manuf, vol. 33, no. 7, pp. 686–700, 2020, doi: 10.1080/0951192X.2018.1550680.
L. Wang, F. Yan, F. Wang, and Z. Li, “FMEA-CM based quantitative risk assessment for process industries—A case study of coal-to-methanol plant in China,” Process Safety and Environmental Protection, vol. 149, pp. 299–311, 2021, doi: 10.1016/j.psep.2020.10.052.
A. K. Singh, S. Avikal, K. C. Nithin Kumar, M. Kumar, and P. Thakura, “A fuzzy-AHP and M - TOPSIS based approach for selection of composite materials used in structural applications,” Mater Today Proc, vol. 26, pp. 3119–3123, 2019, doi: 10.1016/j.matpr.2020.02.644.
Y. Zhao, H. Su, J. Wan, D. Feng, X. Gou, and B. Yu, “Complementarity Evaluation Index System and Method of Multiple Power Sources,” in 2020 IEEE Student Conference on Electric Machines and Systems, SCEMS 2020, 2020, pp. 200–206. doi: 10.1109/SCEMS48876.2020.9352432.
L. Lv, Z. Deng, H. Meng, T. Liu, and L. Wan, “A multi-objective decision-making method for machining process plan and an application,” J Clean Prod, vol. 260, p. 121072, 2020, doi: 10.1016/j.jclepro.2020.121072.
X. Zhang, J. Lu, and Y. Peng, “Hybrid MCDM Model for Location of Logistics Hub: A Case in China under the Belt and Road Initiative,” IEEE Access, vol. 9, pp. 41227–41245, 2021, doi: 10.1109/ACCESS.2021.3065100.
J. Liu, W. Liu, L. Jin, T. Tu, and Y. Ding, “A performance evaluation framework of electricity markets in China,” in Proceedings - 2020 5th Asia Conference on Power and Electrical Engineering, ACPEE 2020, 2020, pp. 1043–1048. doi: 10.1109/ACPEE48638.2020.9136486.
Y. Deng et al., “Thermo-chemical water splitting: Selection of priority reversible redox reactions by multi-attribute decision making,” Renew Energy, vol. 170, pp. 800–810, 2021, doi: 10.1016/j.renene.2021.02.009.
M. Lin, Z. Chen, Z. Xu, X. Gou, and F. Herrera, “Score function based on concentration degree for probabilistic linguistic term sets: An application to TOPSIS and VIKOR,” Inf Sci (N Y), vol. 551, pp. 270–290, 2021, doi: 10.1016/j.ins.2020.10.061.
R. Ran and B. J. Wang, “Combining grey relational analysis and TOPSIS concepts for evaluating the technical innovation capability of high technology enterprises with fuzzy information,” Journal of Intelligent and Fuzzy Systems, vol. 29, no. 4, pp. 1301–1309, 2015, doi: 10.3233/IFS-141380.
J. S. Chiang, P. L. Wu, S. D. Chiang, T. J. Chang, S. T. Chang, and K. L. Wen, “Introduction to Grey System Theory,” Citeseer, vol. 1, pp. 1–24, 1998.
H. A. Alsattar, A. A. Zaidan, and B. B. Zaidan, “Novel meta-heuristic bald eagle search optimisation algorithm,” Artif Intell Rev, vol. 53, no. 3, pp. 2237–2264, Mar. 2020, doi: 10.1007/s10462-019-09732-5.
A. Alnoor et al., “Toward a Sustainable Transportation Industry: Oil Company Benchmarking based on the Extension of Linear Diophantine Fuzzy Rough Sets and Multi-criteria Decision-Making Methods,” IEEE Transactions on Fuzzy Systems, vol. Under Revi, 2022, doi: 10.1109/TFUZZ.2022.3182778.
R. T. Mohammed et al., “Determining Importance of Many-Objective Optimisation Competitive Algorithms Evaluation Criteria Based on a Novel Fuzzy-Weighted Zero-Inconsistency Method,” Int J Inf Technol Decis Mak, Mar. 2021, doi: 10.1142/S0219622021500140.
M. M. Salih, B. B. Zaidan, and A. A. Zaidan, “Fuzzy decision by opinion score method,” Applied Soft Computing Journal, vol. 96, 2020, doi: 10.1016/j.asoc.2020.106595.
E. Krishnan et al., “Interval type 2 trapezoidal-fuzzy weighted with zero inconsistency combined with VIKOR for evaluating smart e-tourism applications,” International Journal of Intelligent Systems, vol. 36, no. 9, pp. 4723–4774, May 2021, doi: 10.1002/int.22489.
F. K. Gündoǧdu and C. Kahraman, “Spherical fuzzy sets and spherical fuzzy TOPSIS method,” Journal of Intelligent and Fuzzy Systems, vol. 36, no. 1, pp. 337–352, 2019, doi: 10.3233/JIFS-181401.
A. A. Vazirani, O. O’Donoghue, D. Brindley, and E. Meinert, “Implementing blockchains for efficient health care: Systematic review,” J Med Internet Res, vol. 21, no. 2, 2019, doi: 10.2196/12439.
J. Xu et al., “Healthchain: A Blockchain-Based Privacy Preserving Scheme for Large-Scale Health Data,” IEEE Internet Things J, vol. 6, no. 5, pp. 8770–8781, 2019, doi: 10.1109/JIOT.2019.2923525.
A. Zhang and X. Lin, “Towards Secure and Privacy-Preserving Data Sharing in e-Health Systems via Consortium Blockchain,” J Med Syst, vol. 42, no. 8, Aug. 2018, doi: 10.1007/s10916-018-0995-5.
M. S. Matell and J. Jacoby, “Is there an optimal number of alternatives for likert scale items? study 1: Reliability and validity,” Educ Psychol Meas, vol. 31, no. 3, pp. 657–674, 1971, doi: 10.1177/001316447103100307.
I. M. Sharaf and E. A. H. A. Khalil, “A spherical fuzzy TODIM approach for green occupational health and safety equipment supplier selection,” International Journal of Management Science and Engineering Management, pp. 1–13, 2020, doi: 10.1080/17509653.2020.1788467.
F. Kutlu Gündoğdu and C. Kahraman, “Optimal Site Selection of Electric Vehicle Charging Station by Using Spherical Fuzzy TOPSIS Method,” in Studies in Fuzziness and Soft Computing, Springer, 2021, pp. 201–216. doi: 10.1007/978-3-030-45461-6_8.
S. Ashraf and S. Abdullah, “Emergency decision support modelling for COVID-19 based on spherical fuzzy information,” International Journal of Intelligent Systems, vol. 35, no. 11, pp. 1601–1645, Nov. 2020, doi: 10.1002/int.22262.
C. C. Sun, “A performance evaluation model by integrating fuzzy AHP and fuzzy TOPSIS methods,” Expert Syst Appl, vol. 37, no. 12, pp. 7745–7754, 2010, doi: 10.1016/j.eswa.2010.04.066.
G. Nagasubramanian, R. K. Sakthivel, R. Patan, A. H. Gandomi, M. Sankayya, and B. Balusamy, “Securing e-health records using keyless signature infrastructure blockchain technology in the cloud,” Neural Comput Appl, vol. 32, no. 3, pp. 639–647, Feb. 2020, doi: 10.1007/s00521-018-3915-1.
X. Liu, Z. Wang, C. Jin, F. Li, and G. Li, “A Blockchain-Based Medical Data Sharing and Protection Scheme,” IEEE Access, vol. 7, pp. 118943–118953, 2019, doi: 10.1109/ACCESS.2019.2937685.
S. Cao, G. Zhang, P. Liu, X. Zhang, and F. Neri, “Cloud-assisted secure eHealth systems for tamper-proofing EHR via blockchain,” 2019. doi: 10.1016/j.ins.2019.02.038.
F. Tang, S. Ma, Y. Xiang, and C. Lin, “An Efficient Authentication Scheme for Blockchain-Based Electronic Health Records,” IEEE Access, vol. 7, pp. 41678–41689, 2019, doi: 10.1109/ACCESS.2019.2904300.
A. Al Omar, M. Z. A. Bhuiyan, A. Basu, S. Kiyomoto, and M. S. Rahman, “Privacy-friendly platform for healthcare data in cloud-based on blockchain environment,” Future Generation Computer Systems, vol. 95, pp. 511–521, 2019, doi: 10.1016/j.future.2018.12.044.
A. Shahnaz, U. Qamar, and A. Khalid, “Using Blockchain for Electronic Health Records,” IEEE Access, vol. 7, pp. 147782–147795, 2019, doi: 10.1109/ACCESS.2019.2946373.
D. C. Nguyen, P. N. Pathirana, M. Ding, and A. Seneviratne, “Blockchain for Secure EHRs Sharing of Mobile Cloud Based E-Health Systems,” IEEE Access, vol. 7, pp. 66792–66806, 2019, doi: 10.1109/ACCESS.2019.2917555.
Y. Wang, A. Zhang, P. Zhang, and H. Wang, “Cloud-Assisted EHR Sharing with Security and Privacy Preservation via Consortium Blockchain,” IEEE Access, vol. 7, pp. 136704–136719, 2019, doi: 10.1109/ACCESS.2019.2943153.
E. Y. Daraghmi, Y. A. Daraghmi, and S. M. Yuan, “MedChain: A design of blockchain-based system for medical records access and permissions management,” IEEE Access, vol. 7, pp. 164595–164613, 2019, doi: 10.1109/ACCESS.2019.2952942.
L. Hang, E. Choi, and D. H. Kim, “A novel EMR integrity management based on a medical blockchain platform in hospital,” Electronics (Switzerland), vol. 8, no. 4, p. 467, Apr. 2019, doi: 10.3390/electronics8040467.
S. Wang, D. Zhang, and Y. Zhang, “Blockchain-Based Personal Health Records Sharing Scheme with Data Integrity Verifiable,” IEEE Access, vol. 7, pp. 102887–102901, 2019, doi: 10.1109/ACCESS.2019.2931531.
A. R. Rajput, Q. Li, M. Taleby Ahvanooey, and I. Masood, “EACMS: Emergency Access Control Management System for Personal Health Record Based on Blockchain,” IEEE Access, vol. 7, pp. 84304–84317, 2019, doi: 10.1109/ACCESS.2019.2917976.
X. Li and M. Tan, “Electronic Certificate Sharing Scheme with Searchable Attribute-Based Encryption on Blockchain,” J Phys Conf Ser, vol. 1757, no. 1, 2021, doi: 10.1088/1742-6596/1757/1/012161.
S. M. Pournaghi, M. Bayat, and Y. Farjami, “MedSBA: a novel and secure scheme to share medical data based on blockchain technology and attribute-based encryption,” J Ambient Intell Humaniz Comput, vol. 11, no. 11, pp. 4613–4641, 2020, doi: 10.1007/s12652-020-01710-y.
L. Chen, W. K. Lee, C. C. Chang, K. K. R. Choo, and N. Zhang, “Blockchain based searchable encryption for electronic health record sharing,” Future Generation Computer Systems, vol. 95, pp. 420–429, 2019, doi: 10.1016/j.future.2019.01.018.
H. Wang and Y. Song, “Secure Cloud-Based EHR System Using Attribute-Based Cryptosystem and Blockchain,” J Med Syst, vol. 42, no. 8, Aug. 2018, doi: 10.1007/s10916-018-0994-6.
T. T. Thwin and S. Vasupongayya, “Blockchain-Based Access Control Model to Preserve Privacy for Personal Health Record Systems,” Security and Communication Networks, vol. 2019, 2019, doi: 10.1155/2019/8315614.
A. Dubovitskaya et al., “ACTION-EHR: Patient-centric blockchain-based electronic health record data management for cancer care,” J Med Internet Res, vol. 22, no. 8, 2020, doi: 10.2196/13598.
Q. Su, R. Zhang, R. Xue, and P. Li, “Revocable Attribute-Based Signature for Blockchain-Based Healthcare System,” IEEE Access, vol. 8, pp. 127884–127896, 2020, doi: 10.1109/ACCESS.2020.3007691.
J. Fu, N. Wang, and Y. Cai, “Privacy-preserving in healthcare blockchain systems based on lightweight message sharing,” Sensors (Switzerland), vol. 20, no. 7, 2020, doi: 10.3390/s20071898.
C. Gan, A. Saini, Q. Zhu, Y. Xiang, and Z. Zhang, “Blockchain-based access control scheme with incentive mechanism for eHealth systems: patient as supervisor,” Multimed Tools Appl, vol. 80, no. 20, pp. 30605–30621, 2021, doi: 10.1007/s11042-020-09322-6.
C. L. Chen, Y. Y. Deng, W. Weng, H. Sun, and M. Zhou, “A blockchain-based secure inter-hospital EMR sharing system,” Applied Sciences (Switzerland), vol. 10, no. 14, 2020, doi: 10.3390/app10144958.
H. Liu, R. G. Crespo, and O. S. Martínez, “Enhancing privacy and data security across healthcare applications using Blockchain and distributed ledger concepts,” Healthcare (Switzerland), vol. 8, no. 3, 2020, doi: 10.3390/healthcare8030243.
A. Margheri, M. Masi, A. Miladi, V. Sassone, and J. Rosenzweig, “Decentralised provenance for healthcare data,” Int J Med Inform, vol. 141, 2020, doi: 10.1016/j.ijmedinf.2020.104197.
T. Benil and J. Jasper, “Cloud based security on outsourcing using blockchain in E-health systems,” Computer Networks, vol. 178, 2020, doi: 10.1016/j.comnet.2020.107344.
H. A. Lee et al., “An architecture and management platform for blockchain-based personal health record exchange: Development and usability study,” J Med Internet Res, vol. 22, no. 6, 2020, doi: 10.2196/16748.
D. Pamucar, M. Yazdani, R. Obradovic, A. Kumar, and M. Torres-Jiménez, “A novel fuzzy hybrid neutrosophic decision-making approach for the resilient supplier selection problem,” International Journal of Intelligent Systems, vol. 35, no. 12, pp. 1934–1986, Dec. 2020, doi: 10.1002/int.22279.
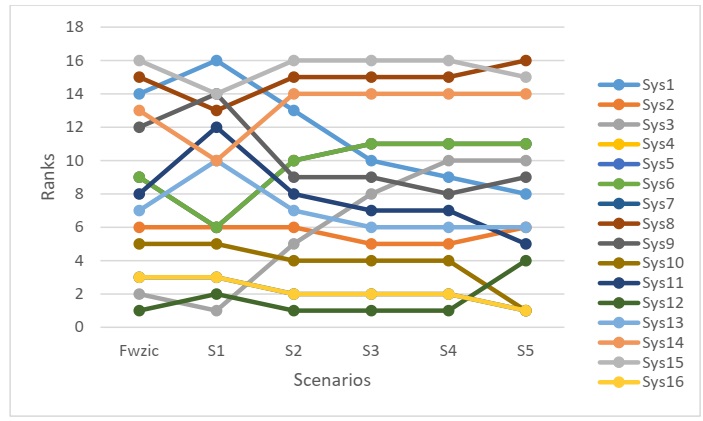
Downloads
Published
How to Cite
Issue
Section
License
Copyright (c) 2023 Sarah Qahtan, Khaironi Yatim, Mohd Hafeez Osman, Hazura Zulzalil, Muhammad Luqman Mahamad Zakaria

This work is licensed under a Creative Commons Attribution 4.0 International License.